AI in loan underwriting: Paving the way for smarter lending
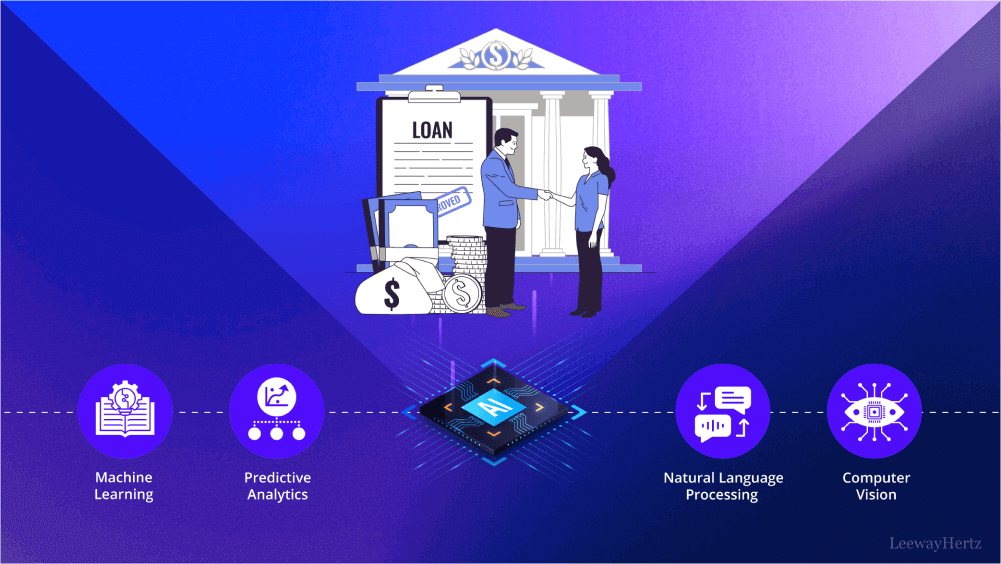
In the intricate landscape of loan underwriting, financial institutions encounter a myriad of challenges that can impede the efficiency and accuracy of the loan sanctioning process. Traditional underwriting methods often grapple with time-consuming tasks, complex data analysis, and the potential for human errors, leading to delays and increased operational costs. The need for a more streamlined and technologically advanced approach has prompted a paradigm shift in the industry, and Artificial Intelligence (AI) has emerged as a transformative force poised to redefine the loan underwriting landscape.
One of the primary hurdles faced by underwriters lies in the exhaustive process of collecting, verifying, and analyzing vast amounts of borrower data. This manual approach not only consumes considerable time but also exposes the lender to the risk of overlooking crucial details or miscalculating risk factors. Furthermore, the evolving nature of financial markets demands a nimble and adaptive underwriting process, a feat that conventional methods struggle to achieve.
Enter AI, a technological powerhouse that harnesses machine learning algorithms to process colossal datasets swiftly and accurately. The integration of AI in loan underwriting introduces automation to mundane tasks, from data collection to risk assessment, significantly reducing the time and resources expended in the process. By learning from historical data patterns and continuously adapting to evolving market dynamics, AI-driven underwriting systems enhance predictive accuracy, allowing financial institutions to make more informed and timely decisions.
This article delves into the transformative role of AI in loan underwriting, exploring how machine learning algorithms streamline data processing, fortify risk assessments, and ultimately expedite loan approval processes. By shedding light on the challenges faced by traditional underwriting methods, the article will unravel the innovative solutions AI brings to the forefront, ushering in a new era of efficiency, precision, and agility in loan sanctioning.
- What is loan underwriting?
- Challenges and limitations in traditional loan underwriting
- A brief overview of AI in loan underwriting
- Use cases of AI in loan underwriting
- How does LeewayHertz’s generative AI platform optimize loan underwriting processes?
- The operational advantages of AI in loan underwriting
- AI technologies transforming loan underwriting
- How does AI/ML-driven automated loan underwriting work?
- Challenges and concerns associated with AI-driven loan underwriting
- The future of AI in loan underwriting
What is loan underwriting?
Loan underwriting is the process by which a financial institution evaluates the creditworthiness of a borrower and assesses the risk associated with lending money to them. During underwriting, the lender reviews various aspects of the borrower’s financial situation, including credit history, income, employment stability, and debt-to-income ratio. The objective is to assess the probability that the borrower can repay the loan, considering their financial profile. Lenders use underwriting to make informed decisions on whether to approve, modify, or deny a loan, as well as to establish the terms and conditions, such as interest rates and loan amounts. The underwriting process is crucial for managing risk and ensuring responsible lending practices.
Challenges and limitations in traditional loan underwriting
Conventional loan underwriting encompasses a thorough evaluation of a borrower’s creditworthiness and their capacity to repay a loan. However, there are many challenges associated with these traditional underwriting methods. Here are some of them:
- Time-consuming process: Traditional underwriting processes can be lengthy, involving significant paperwork and manual review of financial documents. This can result in delays, making it challenging for borrowers to obtain funds quickly.
- High cost: The manual nature of traditional underwriting processes contributes to high operating costs for lenders. This can be a limiting factor for both borrowers and lenders, as it may result in higher interest rates or fees for borrowers and lower profit margins for lenders.
- Limited data sources: Traditional underwriting methods often rely on a limited set of financial data, such as credit scores and income statements. This might not offer a holistic view of a borrower’s financial well-being and capacity to repay a loan, particularly for those with limited credit histories or fluctuating income.
- Subjectivity: Human judgment plays a pivotal role in traditional underwriting, which can introduce subjectivity and inconsistency in decision-making. This may lead to unfair outcomes or biased decisions.
- Risk of fraud: Manual underwriting processes may be susceptible to fraudulent activities, as it can be challenging to thoroughly verify the authenticity of all submitted documents.
- Inflexibility: Traditional underwriting methods may not adapt well to changing economic conditions or unique financial situations. This lack of flexibility can be a limitation in assessing unconventional borrowers or those facing temporary financial challenges.
- Inability to assess non-traditional income sources: Traditional underwriting methods may struggle to accurately assess income from non-traditional sources, such as freelancing, gig work, or rental income. This limitation can impact the ability of some individuals to qualify for loans.
- Limited use of technology: Many traditional underwriting processes still rely on outdated systems and manual data entry. The limited use of technology can hinder efficiency, accuracy, and the ability to incorporate advanced analytics and machine learning.
To address these challenges, the financial industry is increasingly exploring and adopting innovative technologies such as AI, machine learning, and alternative data sources to enhance the efficiency and effectiveness of loan underwriting processes. These advancements aim to streamline operations, reduce costs, and provide a more accurate assessment of a borrower’s creditworthiness.
Launch your project with LeewayHertz!
Enhance your underwriting processes with AI integration. Collaborate with LeewayHertz for custom solutions, ensuring heightened efficiency and accuracy.
A brief overview of AI in loan underwriting
Recognizing the transformative impact of artificial intelligence on the traditional lending landscape is essential in understanding AI in loan underwriting. AI is transforming the underwriting process by harnessing advanced machine learning algorithms and data analytics, facilitating more informed and efficient credit decisions. In contrast to traditional methods, AI-enabled underwriting rapidly analyzes vast datasets, incorporating alternative sources like social media activity, transaction histories, and behavioral patterns. This capability enables a more thorough and nuanced evaluation of a borrower’s creditworthiness, proving especially advantageous for individuals with limited credit histories. Furthermore, AI-driven models exhibit adaptability to changing economic conditions, enhance accuracy, and minimize human bias in decision-making. The integration of AI in loan underwriting not only streamlines processes but also promotes greater financial inclusion by providing a more holistic assessment of borrowers.
Use cases of AI in loan underwriting
AI plays a significant role in transforming various industries, and loan underwriting is no exception. Here are some key use cases of AI in loan underwriting:
Creditworthiness assessment
In the dynamic landscape of commercial lending, the traditional reliance on robust credit scores is evolving with the adoption of automated credit decisioning systems, exemplifying a key use case for AI in loan underwriting. Contemporary lenders are departing from conventional practices, implementing alternative scoring models powered by AI to evaluate customers’ creditworthiness seamlessly. This transformative approach involves efficiently assessing credit scores against predefined standards, utilizing customer bureau data and AI-based credit scoring to ascertain eligibility. Eligible customers are presented with personalized credit offers, and upon acceptance, the streamlined process continues through automated KYC and digital client onboarding. This integration of AI not only expedites the credit assessment but also ensures rapid loan disbursal, addressing the growing demand for a faster and more responsive lending ecosystem. This use case stands as a testament to ongoing innovation and investment in commercial lending, aligning with the contemporary expectation for a swift and efficient credit assessment and disbursal procedure precisely when customers need it.
Elevating customer experience
In banking and credit lending, the integration of AI goes beyond traditional roles, transforming institutions into financial allies for their account holders. Beyond offering standard financial products, such as loans and instruments, banks are leveraging AI to craft a more enriching customer experience. AI’s role begins with the creation of detailed and data-rich customer profiles derived from the meticulous analysis of transaction line items on current accounts or card statements.
Beyond the typical B2B engagement, banks step into the realm of personalized financial companionship. This evolution allows them to provide tailored advice on savings, significant purchases, financial literacy guidance, and pivotal decision-making support. By harnessing AI, banks not only enhance their traditional offerings but also add substantial value to customers’ lives. Digital lending platforms leverage data to extend beyond financial transactions, enabling banks to notify specific customers about exclusive deals and offers tied to their credit reputation. This proactive approach not only cultivates a more experiential banking environment but also streamlines loan operations, forging a seamless and personalized journey for customers.
Streamlined loan processing
The integration of AI in loan underwriting is redefining commercial lending, offering a seamless and digitally-driven experience akin to personal loan applications. While certain regulatory constraints still impact the complete digitization of lending, such as mortgage loans and payments, there is a growing push to automate at least 95% of manual underwriting decisions across various banking domains.
In the domain of SME lending, both traditional banks and fintechs are prioritizing digitization, albeit with caution, in the corporate lending sphere. Rather than a complete overhaul of customer experiences, banks are incrementally refining standard processes, exemplified by the digitization of credit proposal papers and the automation of annual reviews to enhance efficiency.
Strategic initiatives are also underway, focusing on aiding corporate transaction approvers by automating low-risk credit renewals. This ensures that valuable manual resources can be directed toward intricate and high-credit-risk deals. AI’s role in automating data aggregation equips relationship managers with instant access to comprehensive data and risk-evaluation scores, incorporating financial and industry performance, market and sentiment analysis, important news, and external factors. This not only streamlines operations but also empowers businesses to navigate lending decisions with precision and agility, marking a significant leap forward in the landscape of commercial loan underwriting.
Data remediation in loan applications
Addressing data quality, specifically the accuracy, completeness, and reliability of personal, financial, and credit-related information in loan applications, is a critical focus area for AI technologies within the domain of loan underwriting. Recognizing this, banks and lending institutions have instituted comprehensive programs that prioritize data measurement, quality analysis, and the remediation of detected issues. However, traditional remediation processes often prove time-consuming, creating substantial backlogs in operations and requiring significant human resources for data scrubbing.
The infusion of AI intervention and technological integration redefines this landscape, introducing more efficient processes for prioritizing and remediating issues on a broader scale. Dedicated funds are allocated for the implementation of AI-integrated programs and the automation of remediation processes. Validated machine learning models are applied to scrutinize inputs, facilitating the development of analytics-driven models that ensure faster and more accurate decisions in the data remediation process. This strategic integration of AI not only expedites data remediation efforts but also enhances precision, offering a transformative approach to handling data quality in the context of loan applications.
Fortifying loan underwriting against fraud
The surge in cybercrimes amid the digital evolution necessitates robust fraud monitoring in loan underwriting, and AI emerges as a pivotal ally in this realm. In the face of identity thefts, phishing scams, ransomware attacks, and data theft becoming prevalent, the integration of AI and blockchain technology stands out as a game-changer.
AI, coupled with blockchain-powered smart contracts, reshapes identity verification for loan borrowers, ensuring both speed and security. These smart contracts monitor and regulate loans throughout their lifecycle, mitigating the risks of defaults, delayed reporting, and unreported loans. AI-driven solutions offer a shared control view of historical records, authenticate financial information and data, and provide shared access and consensus, minimizing the scope for fraudulent activities. This not only ensures efficient governance but also enhances transparency in the underwriting process. As banks and companies embrace an “AI-first” approach, the foundational importance of a sustainable and sturdy technological backbone cannot be overstated. In the quest for rapid technological advancements, the risk of poor security and fraud looms large. Therefore, rigorous security testing becomes imperative, safeguarding against potential threats and vulnerabilities before innovative AI solutions are deployed for the greater good.
Data extraction through OCR in loan underwriting
Optical Character Recognition (OCR) emerges as a specialized solution, decoding textual information within images and facilitating a transformative approach to data extraction. OCR seamlessly converts text-containing images into computer-readable characters, shaping the future course of action for financial institutions. Banks and commercial lending firms leverage OCR to extract crucial data from physical documents such as cheques, encompassing account information, handwritten amounts, and signatures for authorization. Loan applications laden with diverse documents and information witness a streamlined data extraction process powered by AI technologies. Deep learning enhances this capability, capturing additional data points through OCR to yield more comprehensive outcomes. This not only expedites data recording and maintenance but also contributes to faster decision-making in the loan underwriting process, underscoring the efficiency gains achievable through the integration of OCR technology.
Loan underwriting through behavioral analytics
Advanced behavioral analytics stands as a transformative tool, reshaping how banks and fintech firms process transactions and gain insights from primary operating accounts. Operational instruments have been developed to meticulously classify transactions into detailed revenue and expense items, while advanced behavioral analytics takes this a step further. By harnessing the power of behavioral analytics, banks and institutes can generate simplified financial statements, evaluate affordability ratios, and conduct real-time analyses of customer and supplier concentrations. The wealth of transactional data not only provides updated insights but also serves as a foundation for substantially richer and more pragmatic data solutions, laying the groundwork for iterative data improvement models. A standardized behavioral analytics model becomes the catalyst for innovation in customer experiences and the enhancement of the lending process, exemplifying how AI-driven insights elevate the efficiency and precision of loan underwriting practices.
By leveraging AI in loan underwriting, financial institutions can enhance efficiency, reduce risks, and make more informed lending decisions, ultimately benefiting both lenders and borrowers.
How does LeewayHertz’s generative AI platform optimize loan underwriting processes?
LeewayHertz’s generative AI platform, ZBrain, plays a transformative role in empowering financial institutions by optimizing loan underwriting processes and facilitating swift, efficient loan approvals. As a comprehensive, enterprise-ready platform, ZBrain empowers businesses to design and implement applications tailored to their specific operational requirements. The platform uses clients’ data, whether in the form of text, images, or documents, to train advanced LLMs like GPT-4, Vicuna, Llama 2, or GPT-NeoX for developing contextually aware applications capable of performing diverse tasks.
Enterprises encounter a multifaceted challenge in loan underwriting, grappling with issues spanning data accuracy, regulatory compliance, risk assessment, fraud prevention, subjective decision-making, meticulous documentation management, and adapting to dynamic economic conditions. ZBrain effectively addresses these challenges through its distinctive feature called “Flow,” which provides an intuitive interface that allows users to create intricate business logic for their apps without the need for coding. Flow’s easy-to-use drag-and-drop interface enables the seamless integration of large language models, prompt templates, and other genAI models into your app’s logic for its easy conceptualization, creation, or modification.
To comprehensively understand how ZBrain Flow works, explore this resource that outlines a range of industry-specific Flow processes. This compilation highlights ZBrain’s adaptability and resilience, showcasing how the platform effectively meets the diverse needs of various industries, ensuring enterprises stay ahead in today’s rapidly evolving business landscape.
ZBrain applications facilitate organizations in elevating their loan underwriting workflows, resulting in heightened efficiency, accelerated decision-making, enhanced accuracy in risk assessment, robust fraud detection, cost reduction, consistent and fair application of underwriting criteria, improved customer experiences, and streamlined compliance management, all while upholding stringent data privacy standards.
Launch your project with LeewayHertz!
Enhance your underwriting processes with AI integration. Collaborate with LeewayHertz for custom solutions, ensuring heightened efficiency and accuracy.
The operational advantages of AI in loan underwriting
The integration of AI in loan underwriting processes brings forth a multitude of benefits, redefining the efficiency, accuracy, and overall effectiveness of the lending industry:
Improved accuracy and risk assessment: AI-powered models leverage extensive datasets to discern patterns and assess risk factors, resulting in heightened accuracy in predicting creditworthiness. This enhanced precision enables financial institutions to make well-informed lending decisions, minimizing the likelihood of defaults.
Personalized decision-making: The customization capabilities of AI in underwriting empower lenders to tailor decisions based on individual borrower profiles. This personalization extends to interest rates and loan terms, optimizing the lending experience for a diverse clientele.
Cost reduction: Through the automation of manual tasks, AI significantly reduces operational costs associated with underwriting processes. This efficiency in resource utilization leads to a more cost-effective and sustainable lending operation.
Adaptability to dynamic conditions: AI’s real-time adaptability to changing economic conditions ensures that underwriting models remain responsive to evolving market trends. The continuous learning mechanisms embedded in AI contribute to a dynamic and agile approach in the face of economic fluctuations.
Enhanced fraud detection: AI employs sophisticated algorithms to identify anomalies and detect potential fraud within loan applications. This heightened capability in fraud prevention contributes to a more secure lending environment, protecting both financial institutions and borrowers.
Streamlined document processing: Leveraging Natural Language Processing (NLP) and Computer Vision, AI streamlines the analysis of unstructured data and documents in loan applications. This not only accelerates document processing but also enhances accuracy, reducing reliance on manual efforts.
Increased objectivity and consistency: AI’s rule-based operation reduces the impact of human bias, fostering greater objectivity in lending decisions. Consistent application of predefined algorithms ensures fairness throughout the decision-making process, promoting a more impartial approach.
Scalability: AI-driven systems demonstrate scalability by efficiently managing large volumes of data and applications. This scalability is particularly valuable for financial institutions dealing with expanding loan portfolios, ensuring operational efficiency as the business grows.
Data-driven insights: AI’s data analytics capabilities generate actionable insights, empowering lenders with valuable information for strategic decision-making. This data-driven intelligence aids financial institutions in identifying trends, refining underwriting strategies, and staying ahead in a competitive market.
Enhanced customer experience: Faster processing times and personalized offerings contribute to an improved customer experience in the lending process. Transparent and efficient underwriting instills trust among borrowers, fostering positive relationships between financial institutions and their clientele.
Regulatory compliance: AI systems can be designed to ensure compliance with regulatory standards, reducing the risk of errors. Automated processes enhance precision in adhering to legal requirements, mitigating regulatory challenges faced by financial institutions.
The incorporation of AI into loan underwriting not only addresses the challenges of traditional methods but also introduces a range of benefits that collectively enhance the effectiveness and customer satisfaction within the lending industry.
AI technologies transforming loan underwriting
AI technologies play a crucial role in modernizing and improving the efficiency of loan underwriting processes. Here are some key AI technologies commonly used in loan underwriting:
Machine Learning (ML)
ML plays a crucial role in the modernization of loan underwriting processes, harnessing advanced algorithms and data analytics. In loan underwriting, ML algorithms are trained on extensive datasets to recognize patterns, assess risk factors, and predict creditworthiness. These algorithms can analyze diverse data points, including credit history, income, and financial behavior, providing a more nuanced evaluation of borrowers. ML facilitates real-time decision-making, enabling financial institutions to streamline the underwriting process, improve accuracy in risk assessment, and adapt dynamically to evolving economic conditions. By continuously learning and evolving, ML models contribute to more informed lending decisions, enhancing efficiency and responsiveness in the ever-changing landscape of loan underwriting.
Predictive analytics
Predictive analytics is a cornerstone of modern loan underwriting, harnessing data-driven insights to enhance decision-making processes. In this context, predictive analytics utilizes historical data and statistical algorithms to anticipate future trends and assess potential risks associated with borrowers. By analyzing patterns and relationships within vast datasets, predictive analytics enables financial institutions to evaluate creditworthiness more accurately, predict default probabilities, and optimize interest rates. This technology empowers lenders to make informed decisions, adapt to changing market conditions, and proactively manage risks. Ultimately, predictive analytics in loan underwriting contributes to a more precise, efficient, and forward-looking approach, aligning financial institutions with the evolving needs of the lending landscape.
Natural Language Processing (NLP)
Natural Language Processing (NLP) plays a transformative role in loan underwriting by enabling the analysis and interpretation of unstructured data, such as text. In this context, NLP algorithms process and understand information from various sources, including loan applications, financial statements, and even social media, to extract valuable insights. NLP assists in automating the extraction of relevant information, improving the speed and accuracy of underwriting processes. By comprehending the nuances of language and context, NLP contributes to more informed risk assessments, enhances due diligence, and streamlines the decision-making workflow in loan underwriting, ultimately fostering a more efficient and sophisticated approach to credit evaluation.
Computer vision
While less commonly applied in loan underwriting compared to other fields, computer vision holds the potential for automating document analysis. In this context, computer vision algorithms can visually interpret and extract information from documents such as bank statements, identification cards, and financial records. This technology aids in digitizing and processing physical documents, reducing manual efforts and improving the speed and accuracy of data extraction. Although its application in loan underwriting is evolving, computer vision has the capacity to enhance document verification processes, contributing to more efficient and reliable assessments of borrower information.
Utilizing these AI technologies enables lenders to streamline underwriting processes, improve accuracy, mitigate risks, and, ultimately, make well-informed decisions when extending credit to borrowers. However, it’s crucial to address ethical considerations, data privacy, and regulatory compliance when implementing AI in loan underwriting.
Launch your project with LeewayHertz!
Enhance your underwriting processes with AI integration. Collaborate with LeewayHertz for custom solutions, ensuring heightened efficiency and accuracy.
How does AI/ML-driven automated loan underwriting work?
Automated loan underwriting empowered by AI/ML operates through a systematic process that optimizes efficiency and accuracy. The workflow typically unfolds as follows:
The initiation point involves the gathering of pertinent information from the borrower. This encompasses a spectrum of data such as credit scores, income details, employment history, and other financial metrics. In the digital age, this data is efficiently collected through electronic means, utilizing document management systems and often incorporating digital signatures or e-signatures.
Data pre-processing
Once the requisite data is collected, a critical pre-processing phase follows. This step ensures that the data is formatted correctly and ready for comprehensive analysis. It may involve cleaning the data, filling in any missing values, and normalizing the dataset. This preparatory step is vital to ensure that the data is suitable for analysis by the subsequent AI and ML algorithms.
Data analysis
The core of the automated underwriting process lies in the utilization of AI and ML algorithms to dissect the collected data. These algorithms scrutinize the information for patterns and indicators of risk, thereby assessing the creditworthiness of the borrower.
Risk assessment
Building on data analysis, AI and ML algorithms, often supported by financial models, determine the risk level associated with the borrower. A risk score is assigned to the individual, acting as a pivotal factor in deciding whether they qualify for a loan.
Decision-making
The automated decision-making system employs the risk score to render a verdict on the loan application. This process can occur in real-time, enabling lenders to promptly communicate the status of the loan application to the borrower. In some instances, robo-advisors come into play, automating the provision of financial advice and recommendations to borrowers.
Validation
An additional layer of assurance is integrated through an automated validation system, which verifies the authenticity of the data provided by borrowers. This step ensures that critical details such as income and employment information are accurate and reliable.
Approval or denial
In the final stage, the system decides to approve or deny the loan. This decision is based on the combined results of data analysis and the outputs from the decision-making system. This automated process enhances efficiency and reduces the turnaround time for loan approvals.
Challenges and concerns associated with AI-driven loan underwriting
AI-driven loan underwriting offers numerous benefits, but it also comes with its share of challenges and concerns that need careful consideration. Here are some key challenges and concerns associated with AI-driven loan underwriting:
Ethical considerations and bias
Ensuring fairness in AI models presents a challenge, as they may unintentionally perpetuate biases inherent in historical data. The concern here is that such biases could lead to disparities in lending outcomes for specific demographics or socioeconomic groups, raising ethical considerations about the impact on inclusivity and fairness in the lending process.
Transparency and explainability
A challenge emerges in the lack of transparency within complex AI models, making it challenging for stakeholders to comprehend how decisions are reached. The concern lies in the potential erosion of trust among borrowers, regulators, and financial institutions when there is a lack of clear explanations for loan decisions. This opacity may hinder wider acceptance and understanding of AI-driven underwriting processes.
Data privacy and security
The use of vast amounts of sensitive personal and financial data introduces a challenge concerning data privacy and security. The concern is that inadequate protection may lead to breaches, risking unauthorized access and potential misuse of sensitive information. This underscores the critical importance of robust data security measures in the adoption of AI for loan underwriting.
Customer perception and trust
The challenge lies in building trust in AI-driven systems, which can be difficult due to concerns about fairness, accountability, and the opacity of algorithms. The concern is that a lack of trust might deter individuals from engaging with AI-driven financial services, posing a potential barrier to the broader adoption of advanced technologies in lending.
Model robustness and validation
Ensuring the robustness of AI models presents a challenge, particularly in scenarios not covered in the training data. The concern is that inadequate model validation may result in inaccurate risk assessments, potentially leading to financial losses for lenders. Rigorous validation processes are essential to instill confidence in the reliability of AI-driven underwriting models.
While the adoption of AI in loan underwriting offers unprecedented opportunities for efficiency and accuracy, it is imperative for financial institutions to acknowledge and address the inherent challenges and concerns. Mitigating ethical considerations, enhancing transparency, and prioritizing data security are pivotal steps in realizing the transformative potential of AI-driven underwriting, ensuring a balanced approach that aligns technological innovation with ethical and regulatory standards.
Launch your project with LeewayHertz!
Enhance your underwriting processes with AI integration. Collaborate with LeewayHertz for custom solutions, ensuring heightened efficiency and accuracy.
The future of AI in loan underwriting
The future of AI in loan underwriting holds exciting prospects as technology continues to evolve and financial institutions embrace innovative solutions. Here are key aspects shaping the future landscape:
Expanded use of alternative data: Financial institutions will increasingly leverage alternative data sources beyond traditional credit history. This may include social media activity, online behavior, and other non-traditional indicators, providing a more comprehensive view of an applicant’s financial behavior.
Continuous regulatory adaptation: Regulatory frameworks will adapt to address the distinct challenges introduced by AI in loan underwriting. Governments and regulatory bodies will collaborate to establish guidelines that strike a balance between harnessing the benefits of AI and safeguarding consumer rights, privacy, and equitable lending practices.
Increased focus on education and awareness: With the increasing reliance on AI, there will be a concerted effort to educate stakeholders, including borrowers, about how AI is used in loan underwriting. Transparent communication and awareness programs will be crucial for building trust in AI-driven financial processes.
Innovations in explainable AI: The development of more sophisticated explainability techniques will address the challenge of understanding complex AI models. This will be crucial in gaining trust from both regulatory bodies and the general public.
Cross-industry collaboration: Financial institutions, technology companies, and regulatory bodies will collaborate to share best practices and address common challenges associated with AI in loan underwriting. This collaborative approach will foster a more robust and ethical implementation of AI technologies.
The future of AI loan underwriting will be characterized by continued innovation, increased transparency, and a careful balance between automation and human expertise. As technology advances and regulatory frameworks adjust, AI will become a pivotal force in shaping a lending ecosystem that is more efficient, personalized, and responsible.
Endnote
The integration of AI in loan underwriting marks a paradigm shift in the financial sector, offering a myriad of benefits. AI’s data analysis capabilities allow for a more comprehensive and nuanced evaluation of borrowers, enabling lenders to make informed decisions with increased speed and accuracy. The automation of routine tasks expedites the underwriting process, reducing manual efforts and allowing financial institutions to allocate resources more efficiently. Moreover, AI-driven algorithms can assess risk factors in real-time, adapting to dynamic economic conditions and enhancing the precision of risk assessments. This not only streamlines the lending process but also contributes to more personalized and fair outcomes. The predictive power of AI models aids in identifying patterns and trends, optimizing decision-making and ultimately improving the overall efficiency of loan underwriting. While challenges exist, the transformative impact of AI in this domain underscores its potential to redefine and elevate the lending landscape, fostering innovation, inclusivity, and a more responsive financial ecosystem.
Elevate your underwriting capabilities by integrating AI. Partner with LeewayHertz for custom solutions that will redefine your loan underwriting processes, driving efficiency, precision, and a competitive edge for your business.
Start a conversation by filling the form
All information will be kept confidential.
Insights
Redefining logistics: The impact of generative AI in supply chains
Incorporating generative AI promises to be a game-changer for supply chain management, propelling it into an era of unprecedented innovation.
Understanding generative AI models: A comprehensive overview
Generative AI is a technology used to generate new and original content. It leverages advanced algorithms and neural networks to autonomously produce outputs that mimic human creativity and decision-making.
From diagnosis to treatment: Exploring the applications of generative AI in healthcare
Generative AI in healthcare refers to the application of generative AI techniques and models in various aspects of the healthcare industry.