The rise of AI in banking and finance industry: Exploring use cases and applications
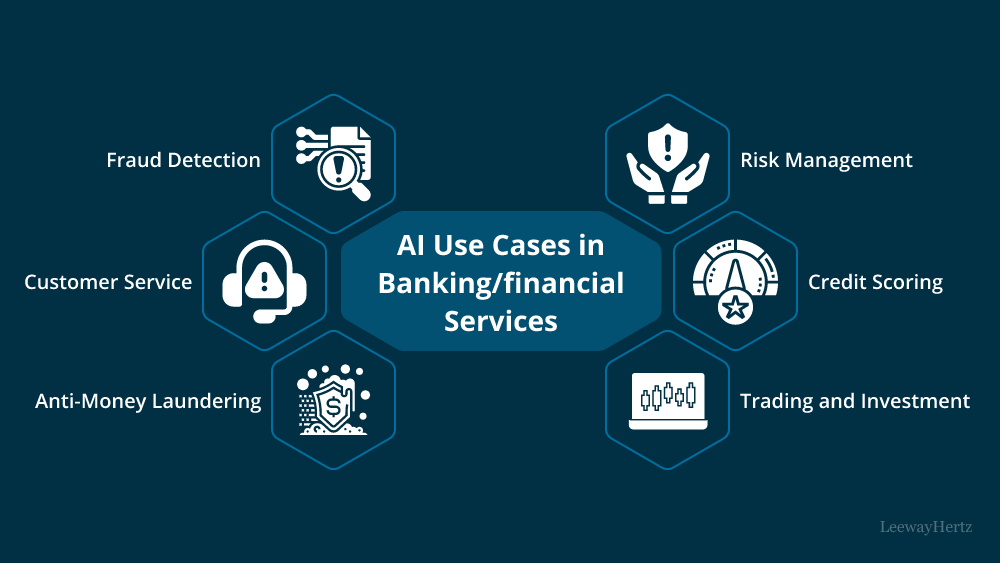
In recent years, the banking industry has undergone many changes, much like other sectors, shifting from traditional practices to ever-expanding digital channels. In this digital age, customers demand more than just convenience – they crave a banking experience that is seamless, swift, and accessible around the clock. Conversational AI has become the linchpin for financial institutions striving to meet and exceed customer expectations. It’s the innovative force driving efficient financial management and resolving banking queries with unprecedented speed and accuracy. Additionally, AI agents are transforming finance with autonomous operations, leveraging advanced technologies to enhance efficiency, improve decision-making, and deliver personalized services, transforming traditional practices and driving innovation in the industry. From monitoring account balances to the intricate processes of credit card applications and loan requests, we find ourselves in an era marked by the presence of intelligent virtual assistants and chatbots. In a financial landscape where time is of the essence, these digital companions empower customers, granting them the capability to autonomously address their financial requirements at any time, around the clock.
This article delves into the diverse use cases and applications of AI in banking and finance, highlighting the benefits and upcoming trends that shape the future of AI in this industry.
- AI’s impact on the banking and finance industry
- AI use cases and applications in the banking and financial services industry
- Fraud detection
- Real-time transaction monitoring
- Automated credit checks
- Chatbots and virtual assistants
- Personalized recommendations
- Analyzing customer behavior
- Analyzing market trends
- Managing investment portfolios
- Automated loan approvals
- Customer segmentation
- Automating risk management
- Competitor analysis
- Streamlining regulatory compliance
- Predictive analytics
- AI-driven contract analysis
- Automated financial report generation
- Identification of upsell & cross-sell opportunities
- Accurate customer churn prediction
- Advanced document processing
- Automated regulatory reporting
- Debt management
- Advanced document processing
- Secured transactions
- Spend category analysis
- Financial robo-advisory
- AI applications and use cases spanning various departments within a financial enterprise
- Important key stakeholders of AI in finance
- How LeewayHertz’s enterprise AI solution transforms banking and finance operations
- How to implement AI solutions in your finance business?
- How to build a financial assistance app?
- Conversational AI in the banking and financial services industry
- Benefits of AI in the banking and finance industry
- Maximizing efficiency in finance and banking: The impact of AI agents
- Challenges and considerations in using AI for finance
- Future of AI in the banking and finance industry
AI’s impact on the banking and finance industry
Artificial Intelligence is an innovative and dynamic technology that has the potential to impact the banking and finance industry significantly. AI encompasses a range of techniques that enable machines to simulate human intelligence and perform tasks with remarkable precision. AI in banking and finance offers various opportunities for process optimization, risk management, and customer engagement. One of the key areas where AI demonstrates its potential is in data analysis. With its ability to process large volumes of structured and unstructured data, AI algorithms can identify patterns, trends, and anomalies that may go unnoticed by human analysts. This data-driven approach enhances decision-making, allowing banks and financial institutions to identify potential risks, predict market trends, and optimize investment strategies. AI also plays a crucial role in customer engagement. By leveraging natural language processing and machine learning, AI-powered chatbots and virtual assistants can interact with customers, providing personalized assistance and support. These intelligent systems can handle routine inquiries, process transactions, and offer tailored recommendations, ultimately enhancing the customer experience and improving satisfaction levels. Moreover, AI can potentially improve security and fraud detection in the banking and finance industry. AI algorithms can analyze vast amounts of data in real time, identifying suspicious activities and potential fraud patterns. This proactive approach helps prevent financial losses and protects both customers and institutions. By incorporating AI, banking and financial institutions can stay competitive in an increasingly digital and data-driven landscape while providing enhanced value to their customers.
AI use cases and applications in the banking and financial services industry
AI redefines the banking and financial services industry by enabling institutions to automate processes, gain insights, and improve customer experiences. Here are some of the use cases and applications of AI in banking and finance:
Fraud detection
Fraud has always been a major concern for banks and financial institutions. Every year, billions of dollars are lost due to fraudulent activities, such as identity theft, credit card fraud, and money laundering. Recently, AI has emerged as a powerful tool to combat fraud. A recent report states that approximately 58 percent of banks reported significant utilization of AI for fraud detection. AI algorithms can analyze vast amounts of data in real time, enabling banks and financial institutions to detect suspicious activity and prevent losses. The enhanced understanding of fraud patterns empowers machine learning models to detect suspicious activities more accurately and effectively. This leads to quicker identification and prevention of fraudulent transactions, reducing the financial losses that institutions might otherwise incur. Timely intervention can save money and protect the institution’s assets and customers’ funds.
Real-time transaction monitoring
In the context of transaction security, AI algorithms excel in real-time pattern recognition and anomaly detection. They scrutinize transaction data to spot patterns that might signify fraudulent activities. For instance, if multiple transactions occur from distinct locations quickly, it could signal an attempt to use a stolen credit card. Likewise, AI algorithms watch spending behaviors, readily identifying sudden spending surges or purchases in unusual categories as potential red flags. Moreover, they analyze the temporal aspects of transactions, assessing factors like timing, frequency, and location to pinpoint suspicious activity.
Automated credit checks
Automating credit checks using AI algorithms is a game-changer for banks and financial institutions. These algorithms can ingest and process vast customer data, encompassing credit history, employment records, financial statements, and more. By tapping into this data trove, they swiftly and accurately assess a customer’s creditworthiness. This assessment involves assigning credit scores based on the data analysis, allowing institutions to make informed lending decisions in a fraction of the time.
AI algorithms also undertake a comprehensive risk assessment, identifying potential hazards tied to an individual’s financial history and behavior. Whether it’s recognizing a history of late payments, high debt levels, or a pattern of loan defaults, these algorithms flag pertinent issues. Furthermore, automation eliminates the need for labor-intensive data entry and analysis, significantly reducing the time and cost associated with traditional credit checks.
AI-powered solutions demonstrate exceptional effectiveness in credit risk management. For instance, the US-based FinTech company Zest AI achieved a remarkable 20% reduction in losses and default rates by leveraging AI for credit risk optimization. AI systems provide early warnings and alerts for potential credit defaults or deteriorating creditworthiness. The use of AI in finance promises transformative impacts on credit allocation and risk assessment, leading to more financial systems.
Chatbots and virtual assistants
AI chatbots provide efficient first-level support by handling routine customer queries and concerns. They can promptly provide information on account balances, transaction history, and account details, freeing human customer service agents to focus on more complex issues. They provide near-instantaneous responses to customer queries by analyzing customer data, such as transaction history and spending patterns, to provide personalized recommendations to customers. Chatbots reduce waiting times and contribute to a more positive customer experience. Moreover, these chatbots are available round-the-clock, ensuring customers can access assistance and information whenever needed, even outside regular business hours. This availability enhances customer satisfaction and engagement.
Personalized recommendations
AI is pivotal in delivering personalized financial planning and recommendations. It accomplishes this by meticulously analyzing an individual’s financial data, encompassing transaction history, income, expenses, savings, and investment patterns. This data-driven approach allows AI to understand the customer’s financial situation comprehensively. Once armed with this information, AI engages in a dialogue with the customer to establish clear financial goals. These objectives are customized to the individual’s unique circumstances and aspirations, whether saving for a buying home, planning for retirement, or investing in education.
Furthermore, AI conducts a thorough risk assessment, determining the customer’s comfort level with investment risks. This critical insight helps shape the recommendations, ensuring they align with the customer’s risk tolerance. AI formulates personalized asset allocation strategies based on established goals and risk profiles. The AI-powered systems recommend investment plans tailored to optimize the customer’s financial position.
Analyzing customer behavior
AI is critical in analyzing customer behavior in the banking and finance sector. Initially, it collects a wealth of data from various sources, including transaction records, account balances, customer demographics, and online interactions. This information is then integrated into a cohesive database, providing a comprehensive view of each customer’s financial profile. AI excels at recognizing patterns and trends through advanced algorithms. It identifies recurring behaviors, such as consistent bill payments, frequent online shopping, or diligent savings habits. Such pattern recognition enables AI to gain insights into individual financial habits and preferences. AI systems adapt as new data is generated, refining their insights and predictions. This dynamic process allows banks and financial institutions to anticipate customer needs, prevent fraud, and enhance customer experience.
Analyzing market trends
AI algorithms can analyze market trends to identify patterns and generate insights. For example, AI can identify patterns in stock prices and predict future trends, helping investors to make informed investment decisions. It can comprehensively understand market trends by analyzing data from various sources, such as news articles, social media, and financial reports. This information can help investors to adjust their investment strategies and minimize potential risks. For instance, if AI predicts an upcoming recession, investors may shift their investments to more defensive sectors to mitigate potential losses.
Managing investment portfolios
AI can also assist in managing investment portfolios by providing insights into portfolio performance and identifying potential risks. For instance, AI can identify stocks that may be underperforming or overvalued, allowing investors to decide whether to sell or hold. AI can also provide recommendations on portfolio rebalancing or asset allocation, helping investors achieve their investment goals. AI algorithms can identify investment opportunities by analyzing market data and identifying undervalued stocks or emerging trends. For example, AI can analyze data from various industries, identify companies with high growth potential, and recommend investment strategies, such as diversification or risk management.
Automated loan approvals
An AI-automated loan approval system is a solution employed by financial institutions to simplify and expedite the loan application process. Through this system, borrowers submit their loan requests electronically, providing essential financial information and personal details. The system then diligently gathers and verifies data from various sources, including credit reports and income statements, ensuring the accuracy of the provided information. The system uses advanced algorithms and credit scoring models to assess the applicant’s creditworthiness by considering factors such as credit history and debt-to-income ratio. This comprehensive risk assessment empowers the system to make informed lending decisions, automatically determining loan approval or rejection and customizing loan terms such as interest rates and repayment schedules, ultimately streamlining the lending process for borrowers and lenders.
Customer segmentation
AI enables customer segmentation in the banking sector by assessing creditworthiness. Higher credit score customers receive tailored loan offerings, such as lower interest rates or increased loan amounts, optimizing incentives for creditworthy individuals. Conversely, those with lower credit scores are presented with more conservative loan terms, enhancing risk management and aligning lending strategies with individual financial profiles. This customization improves precision in targeting, fostering a more personalized and efficient lending experience for diverse customer segments.
Automating risk management
AI can also automate risk management by analyzing data from various sources, such as news articles, financial reports etc., to identify potential risks. For example, AI can analyze news articles about a particular industry or company and identify potential risks, such as legal issues or reputational damage. Banks and financial institutions can proactively identify and mitigate potential compliance issues by automating risk management.
Competitor analysis
Competitor analysis in the banking and finance sector empowers institutions to gain a strategic advantage by rapidly processing vast datasets. Machine learning algorithms analyze competitors’ market positions, product offerings, and customer behaviors, providing valuable insights. Through sentiment analysis on social media and news sources, AI identifies emerging trends and sentiments, enabling financial institutions to adapt swiftly to market dynamics. This data-driven approach enhances decision-making, fosters innovation, and positions organizations to respond to competitive challenges in this rapidly evolving industry proactively.
Streamlining regulatory compliance
By harnessing AI, financial institutions can streamline compliance checks and reporting, reducing the time and costs associated with these critical tasks. AI-driven systems effectively monitor various data sources to ensure adherence to data privacy regulations and anti-money laundering (AML) protocols. They excel in detecting anomalies in financial transactions, improving Know Your Customer (KYC) verification, and continuously monitoring customer behavior for signs of fraud. AI’s role in regulatory compliance minimizes non-compliance risk and bolsters customer confidence by demonstrating a commitment to data protection and financial integrity.
Predictive analytics
External global factors, such as currency fluctuations, natural disasters, or political unrest, can significantly influence the banking and financial sectors. In times of high volatility, making prudent business decisions becomes paramount. AI in the banking industry provides analytical insights that offer a more comprehensive understanding of future scenarios, aiding in proactive preparedness and timely decision-making.
AI-driven contract analysis
AI-driven contract analysis is transforming the banking and finance sector by automating and expediting the traditionally time-consuming process of contract review. Using advanced natural language processing (NLP) algorithms, AI systems can swiftly analyze complex legal documents, identifying key terms, risks, and obligations. This not only reduces the burden on legal teams but also enhances accuracy and ensures compliance with regulatory requirements. By harnessing AI for contract analysis, financial institutions can streamline operations, mitigate risks, and make more informed decisions, ultimately improving efficiency and regulatory adherence in the contract management lifecycle.
Furthermore, AI plays a pivotal role in the financial sector by identifying potentially risky loan applications by assessing a client’s likelihood of repayment failure. This predictive analysis evaluates historical behavioral patterns and smartphone data to anticipate future behavior.
Automated financial report generation
AI is playing an increasingly vital role in automating financial report generation within the finance industry. AI-powered systems excel in analyzing extensive financial data from multiple sources, streamlining the report generation process for businesses. By leveraging AI in financial services, companies can extract pertinent information, validate data accuracy, and produce comprehensive and error-free financial reports efficiently. A notable AI application in finance is the automation of regulatory reporting, where financial institutions must comply with complex regulations and submit accurate reports to regulatory authorities. AI implementation simplifies this process by automatically extracting data, performing necessary calculations, and generating reports that align with regulatory standards.
Moreover, AI facilitates the production of real-time financial reports by swiftly analyzing data, providing stakeholders with up-to-date information crucial for informed decision-making. The integration of AI in finance has significantly transformed financial report generation, optimizing data processing, analysis, and utilization practices within the industry.
Identification of upsell & cross-sell opportunities
Banks and financial institutions utilize AI to identify unmet customer needs, allowing them to pinpoint upsell and cross-sell opportunities accurately. By leveraging AI-driven insights from CRM data, these institutions can offer personalized products and services tailored to specific customer needs, thereby enhancing customer satisfaction and boosting revenue streams. Proactively identifying these opportunities enables banks to deepen customer relationships, drive product adoption, and achieve sustainable growth in today’s competitive market landscape.
Accurate customer churn prediction
AI models play a critical role in customer churn prediction, analyzing patterns in customer behaviors to forecast which customers are likely to churn in the near future. By leveraging these insights, banks and financial institutions can proactively identify at-risk customers and take targeted actions to prevent churn. Understanding the reasons behind customer attrition enables institutions to implement personalized retention strategies, fostering customer loyalty and optimizing customer lifetime value. Through AI-driven churn prediction, institutions can enhance customer relationships, reduce churn rates, and ultimately strengthen their competitive position in the market.
Advanced document processing
AI efficiently processes a variety of financial documents, including statements, receipts, contracts, and agreements, extracting valuable insights and information from each document. AI automates the extraction, interpretation, and processing of information embedded within these documents, significantly enhancing efficiency, accuracy, and decision-making processes within financial institutions. By leveraging AI for document processing, businesses can streamline workflows, reduce manual effort, mitigate errors, and derive actionable insights from complex financial data, ultimately improving operational effectiveness and driving strategic decision-making.
Automated regulatory reporting
Within the highly regulated financial industry, compliance with regulatory requirements is paramount. AI technologies offer solutions to automate the often laborious and error-prone process of generating and submitting regulatory reports. By employing AI algorithms, financial institutions can streamline data collection, analysis, and reporting, enhancing both efficiency and accuracy. This not only saves time and resources but also ensures compliance with complex regulatory frameworks, reducing the risk of penalties and regulatory breaches.
Debt management
Debt collection and management pose significant challenges for businesses, particularly in the face of rising debt levels and economic uncertainties. AI-driven solutions offer innovative approaches to automate and optimize debt collection processes, leveraging advanced analytics, machine learning, and behavioral science techniques. By analyzing customer data and payment behavior, AI systems can identify the most effective collection strategies, prioritize accounts for follow-up, and negotiate repayment terms with delinquent customers. These AI-powered debt management solutions not only improve collection rates but also enhance customer satisfaction by providing personalized and empathetic support to borrowers in financial distress. Overall, AI offers a promising opportunity to transform debt management practices, making them more efficient, effective, and customer-centric.
Customer churn prediction
AI models analyze customer behavior and transaction data to predict churn rates accurately. By identifying patterns and trends indicative of customer dissatisfaction or disengagement, banks can take proactive measures to retain at-risk customers. AI-powered churn prediction models enable banks to segment their customer base, identify high-value customers, and tailor retention strategies to meet their specific needs and preferences. By reducing churn rates, banks can improve customer retention, enhance profitability, and maintain a competitive edge in the market.
Secured transactions
Data security is a top priority for banks and financial institutions, given the sensitive nature of financial transactions and customer information. AI technologies play a pivotal role in enhancing security measures by employing advanced authentication methods such as biometric recognition, voice and face recognition, and blockchain encryption. Major FinTech companies like Adyen, Payoneer, Paypal, and Stripe are at the forefront of integrating AI-driven security solutions to safeguard against fraudulent activities and data breaches. By leveraging AI, these companies can detect and prevent unauthorized access to sensitive information, ensuring trust and confidence among customers.
Spend category analysis
AI enables financial institutions to conduct detailed analyses of spending categories, providing valuable insights into consumer behavior and market trends. By leveraging machine learning algorithms, banks can analyze transaction data to identify patterns, trends, and anomalies in spending behavior. This information can help banks develop targeted marketing campaigns, tailor financial products to customer needs, and optimize pricing strategies to maximize profitability.
Financial robo-advisory
Robo-advisors have emerged as popular tools for providing automated investment advice to clients. These virtual financial consultants leverage AI algorithms to manage investment portfolios, offering personalized recommendations tailored to individual financial goals and risk profiles. Robo-advisors not only automate portfolio management but also provide additional services such as tax optimization, access to human advisors, and diversified investment options. By utilizing AI, platforms like Wealthfront and Betterment have disrupted the traditional wealth management industry, offering cost-effective and accessible investment solutions to a wider audience.
AI applications and use cases spanning various departments within a financial enterprise
As technology advances, the use of AI in banking and other financial sectors is enormously growing. Here are some key applications of AI spanning various departments within a financial institution:
Customer service
Customer service is a crucial aspect of the banking and financial services industry. Providing excellent customer service can improve customer satisfaction, retention, and loyalty. With the advent of AI, banks and financial institutions are using chatbots and virtual assistants to provide 24/7 support to their customers. AI-powered chatbots can handle customer queries, provide personalized recommendations, and even complete transactions on behalf of customers.
Retail banking
The retail banking department’s crucial responsibility lies in managing customer accounts. This includes the opening, maintaining, and overseeing of a wide array of accounts, such as savings accounts, certificates of deposit (CDs) and retirement accounts. Accurately maintaining customer account data is paramount for the department’s seamless operations. The incorporation of AI-powered biometric authentication, including facial recognition or fingerprint scanning, has been seamlessly integrated into the account opening procedure. AI-driven systems automatically extract essential details from customer documents and applications, such as identification cards or utility bills. This eliminates the need for manual data entry, reducing errors and saving time during the account opening process.
Commercial banking
Artificial Intelligence can significantly enhance various aspects of commercial banking functions, including credit lines, financial solutions, risk assessment, and relationship management. AI-powered algorithms can analyze vast amounts of data to assess the creditworthiness of business clients more accurately. They can consider traditional financial data and alternative data sources, such as social media activity and online reviews of their business, to provide a more comprehensive credit assessment. AI can help banks dynamically adjust credit limits for business clients based on real-time financial data and risk factors. This flexibility ensures that businesses have access to the appropriate amount of funds when they need it. AI-driven automation can streamline routine financial tasks, such as cash flow forecasting and reconciliation, reducing the administrative burden on businesses and allowing them to focus on strategic decision-making.
Investment banking
AI in investment banking includes corporate finance, mergers and acquisitions, capital markets, and investment portfolio management. It empowers these financial activities by leveraging data analytics, risk assessment, algorithmic trading, and personalized client interactions. AI enhances decision-making precision, streamlines operational processes, optimizes investment strategies, and fosters stronger client relationships while maintaining regulatory compliance as a cornerstone of its contributions to the financial industry.
Legal Compliance
Artificial Intelligence significantly aids financial institutions’ regulatory compliance and legal departments by automating regulatory monitoring, risk assessment, and due diligence processes, enhancing efficiency and accuracy. AI-powered algorithms analyze vast datasets to identify potential compliance breaches, detect irregularities, and assess real-time risks. Additionally, AI-driven legal research tools streamline interpreting and staying updated on complex regulatory changes, ensuring timely compliance adjustments. AI also facilitates risk analysis, ultimately reducing operational burdens, minimizing legal risks, and enabling proactive compliance measures.
Revenue operations
Artificial Intelligence (AI) is fundamentally transforming Revenue Operations (RevOps) by optimizing financial planning, process efficiency, and customer revenue management in the finance sector. AI-powered algorithms analyze vast datasets to enhance forecasting accuracy, identify risks, and provide real-time insights into revenue performance, empowering finance teams to make data-driven decisions swiftly. Automation of routine tasks through AI enhances operational efficiency, reducing manual efforts and minimizing errors. Moreover, AI-driven customer segmentation, churn prediction, and personalized recommendations enable tailored revenue management strategies, enhancing customer engagement and retention rates. Additionally, AI-driven insights facilitate dynamic pricing strategies, market intelligence gathering, and automated compliance management, empowering finance teams to respond promptly to market shifts and regulatory demands. Ultimately, AI in RevOps for finance optimizes revenue streams, informs growth strategies, and drives operational efficiency, positioning organizations to excel in a dynamic financial landscape.
Operations
Operational processes such as debt collection, procure-to-pay, and commercial banking account reconciliation can be significantly enhanced through the implementation of AI technologies. In debt collection, AI offers the potential to streamline processes and ensure compliance, addressing common complaints such as attempts to collect debts not owed. Likewise, in procure-to-pay, AI-driven invoice capture technologies can automate systems, improve billing efficiency, and accelerate payment reminders, ultimately reducing manual errors and costs. In commercial banking, AI facilitates the extraction and comparison of data from bank statements, expediting reconciliation processes and minimizing disruptive errors. These AI-driven solutions hold promise for optimizing operational efficiency and enhancing financial services across various sectors.
Audit and compliance
Banks are increasingly challenged by the rising threats of cyber and data breaches, compounded by the complexities of recovering losses from fraudulent activities, as highlighted in a KPMG survey. To address these issues, AI innovations emerge as powerful solutions, augmenting fraud detection capabilities, fortifying system security, and alleviating operational burdens. DZ Bank’s case study exemplifies the transformative impact of AI, dramatically reducing security team workloads by 36-fold. Also, in regulatory compliance, AI efficiently scans legal documents, ensuring adherence to mandates while minimizing manual intervention. AI-driven travel and expense management optimizes expenditure validation processes, reinforcing compliance with VAT deduction and income tax regulations while mitigating risks associated with fraud and payroll taxation.
Lending
In lending operations, document capture technologies offer financial institutions the opportunity to automate credit applicant evaluations, minimizing manual review processes of financial documents such as payslips and invoices. AI algorithms streamline data extraction, reducing human intervention and enabling faster and more accurate credit application assessments. By capturing relevant data from borrower companies’ financial documents like annual reports and cash flow statements, banks can enhance credit evaluation accuracy and expedite lending services. AI-enabled credit scoring systems leverage predictive models to assess creditworthiness swiftly and efficiently, resulting in faster decision-making and reduced regulatory costs. For instance, Discover Financial Services has achieved a tenfold acceleration in credit assessment processes and a more comprehensive borrower assessment by employing AI technologies in credit evaluation.
Important stakeholders of AI in finance
In the financial sector, various stakeholders play pivotal roles in the adoption, implementation, and regulation of artificial intelligence (AI) technologies. These stakeholders collaborate to ensure the effective and ethical integration of AI into financial services. Here are the key stakeholders and their respective roles:
1. Auditors and internal control teams
- Role: Responsible for assessing the effectiveness, reliability, and compliance of AI systems within financial organizations.
- Tasks: Conduct audits to identify potential risks, ensure data accuracy, and verify regulatory compliance in AI-driven processes.
2. Chief Information Officers (CIOs) and Chief Technology Officers (CTOs)
- Role: Oversee the technical infrastructure and strategic direction of AI implementation within financial institutions.
- Responsibilities: Make decisions regarding AI adoption, deployment, security protocols, and infrastructure investments to optimize operational efficiency.
3. Customers
- Role: End-users of AI-driven financial services and applications.
- Expectations: Expect AI-driven financial services and applications to provide seamless, personalized experiences that foster trust in technology for efficient banking transactions, personalized investment advice, and responsive customer support.
4. Developers and data scientists
- Role: Design, develop, and maintain AI algorithms and models used in financial applications.
- Tasks: Ensure the accuracy, reliability, and scalability of AI solutions, addressing challenges such as data quality and algorithm transparency.
5. Ethics and diversity officers
- Role: Safeguard against bias and promote fairness and inclusivity in AI deployment and decision-making.
- Responsibilities: Monitor AI systems for ethical implications, diversity gaps, and potential biases to ensure equitable outcomes and user trust.
6. Executives and board of directors
- Role: Make strategic decisions regarding AI initiatives and investments based on business objectives and industry trends.
- Responsibilities: Provide leadership and governance to steer AI strategies, allocate resources, and oversee risk management practices.
7. Financial organizations (Banks, Investment Firms, etc.)
- Role: Deploy AI technologies to enhance operational efficiency, risk management, fraud detection, customer service, and investment strategies.
- Applications: Utilize AI for credit scoring, algorithmic trading, regulatory compliance, and personalized financial advice.
8. Legal and compliance teams
- Role: Ensure that AI applications comply with regulatory frameworks, data protection laws, and industry standards.
- Tasks: Collaborate with regulators to address legal implications, privacy concerns, and ethical considerations associated with AI adoption.
9. Risk Management Teams
- Role: Evaluate and mitigate risks associated with AI implementation and usage in financial operations.
- Responsibilities: Monitor AI-driven processes to identify potential risks, ensure data security, and optimize risk-return profiles.
Collaboration among these diverse stakeholders is essential for leveraging the full potential of AI in finance while addressing challenges related to data privacy, ethics, regulatory compliance, and customer trust. By working together, these stakeholders contribute to the responsible and innovative integration of AI technologies that drive efficiency and transform financial services.
How LeewayHertz’s enterprise AI solution transforms banking and finance operations
LeewayHertz’s generative AI platform, ZBrain, plays a transformative role in optimizing banking and finance operations. As a comprehensive, enterprise-ready platform, ZBrain empowers businesses to develop and implement applications tailored to their specific operational requirements. The platform uses clients’ data, whether in the form of text, images, or documents, to train advanced LLMs like GPT-4, Vicuna, Llama 2, or GPT-NeoX for developing contextually aware applications capable of performing diverse tasks.
In the financial industry, challenges such as complex risk assessment, time-consuming data analysis, and personalized customer interactions are prevalent. ZBrain effectively addresses these challenges through its distinctive feature called “Flow,” which provides an intuitive interface that allows users to create intricate business logic for their apps without the need for coding. Flow’s easy-to-use drag-and-drop interface enables the seamless integration of prompt templates, large language models, and other generative AI models into your app’s logic for its easy conceptualization, creation, or modification.
ZBrain apps convert intricate financial data into actionable insights, helping banking and finance businesses boost operational efficiency, lower error rates, improve overall service quality, and address key challenges that they face. Some challenges that ZBrain helps banking and finance institutions address are-
AI-driven financial product recommendations
ZBrain enables the creation of automated AI-driven systems that significantly minimize the time and resources needed to generate personalized product recommendations. This heightened efficiency enables financial institutions to provide more timely and accurate suggestions, ultimately enhancing customer satisfaction and cultivating stronger loyalty. Experience the paradigm shift in decision-making that ZBrain brings about by seamlessly merging efficiency with personalization, revolutionizing how financial institutions engage and serve their customers.
AI-powered financial risk management
ZBrain plays a pivotal role in transforming risk management within the finance and banking sector by automating labor-intensive tasks and enhancing the efficiency of risk assessment processes. The significant time savings from automated risk mitigation methods enable financial institutions to promptly address emerging risks, make well-informed decisions, and implement robust mitigation strategies. Explore the capabilities of ZBrain in empowering institutions to elevate their risk management practices, ensuring the stability and success of financial operations.
AI-powered operational efficiency analysis
ZBrain significantly enhances operational efficiency within the finance and banking sector by providing financial professionals with data-driven decision-making capabilities for vital operational tasks. With ZBrain apps, finance and banking professionals can swiftly make data-driven decisions, achieving reduced operational costs and optimized customer interactions. Embrace ZBrain to unlock unparalleled operational efficiency and enhance the success of your organization.
AI-driven contract analysis
ZBrain transforms contract management in the finance and banking sectors through automated contract analysis, dramatically reducing the time and resources required. This enables finance and banking professionals to expedite contract evaluations precisely, facilitating informed decision-making, streamlined compliance, and effective risk management. Leverage the power of ZBrain to elevate contract management and drive financial optimization.
AI-driven customer churn analysis
Through ZBrain’s automated customer churn analysis, financial institutions can proactively identify at-risk customers, taking timely actions to reduce churn rates. The streamlined process not only yields considerable time and cost savings but, more significantly, elevates customer retention. The ability to make data-driven decisions and offer personalized solutions strengthens customer relationships, fostering long-term loyalty and contributing to the success and sustainability of banking and finance businesses. Embrace the power of ZBrain to enhance customer service, retain valuable customers, and maintain a competitive edge in the industry.
AI-powered competitor analysis
ZBrain is pivotal in transforming competitor analysis within the finance and banking industry by substantially reducing the time and effort required. With ZBrain, financial institutions can make well-informed strategic decisions, stay ahead of market trends, and navigate regulatory challenges swiftly. Leverage the power of ZBrain to gain a competitive edge and enhance your organization’s success in the dynamic landscape of finance and banking.
How to implement AI solutions in your finance business?
Implementing AI in banking and finance involves several steps to ensure the successful integration and optimization of artificial intelligence technologies. Here’s a general guide on how to implement AI in this sector:
- Define objectives and use cases:
- Clearly outline the goals and objectives of integrating AI into your banking and finance operations.
- Identify specific use cases such as fraud detection, customer service automation, risk management, and personalized financial services.
2. Data collection and preparation:
- Collect and systematically arrange pertinent data from diverse origins, encompassing transaction records, customer details, and market information.
- Ensure data quality and accuracy, as AI models’ success depends on the input data quality.
3. Infrastructure and technology stack:
- Invest in a robust IT infrastructure capable of handling AI workloads.
- Choose suitable AI technologies and frameworks based on the identified use cases, such as machine learning libraries, natural language processing tools, and data processing platforms.
4. AI model development and training:
- Engage data scientists and AI specialists to develop and train ML models based on the chosen use cases.
- Train models using historical data and continually refine them as new data becomes available.
5. Integration with existing systems:
- Integrate AI solutions seamlessly with existing banking and financial systems, like core banking systems, CRM platforms, and transaction processing systems.
- Ensure interoperability and data flow between AI components and legacy systems.
6. Testing and validation:
- Ensure rigorous testing of AI models within a controlled environment to validate their precision and efficacy.
- Test for various scenarios, including edge cases and potential challenges that may arise in real-world situations.
7. Monitoring and maintenance:
- Establish real-time monitoring systems to observe and assess the performance metrics of AI models actively.
- Establish protocols for regular maintenance, updates, and retraining of models to adapt to evolving patterns and changes in the financial landscape.
8. Feedback loop and continuous improvement:
- Establish a feedback loop to collect insights from users and monitor the impact of AI on business processes.Use this feedback to improve and optimize AI models and their implementations iteratively.
LeewayHertz is a leading AI development company specializing in creating tailored solutions for banking and finance businesses. Leveraging a demonstrated history of success in crafting AI applications, LeewayHertz provides extensive expertise to elevate and optimize your business operations. From fraud detection algorithms and personalized financial advisory tools to automated loan processing systems, our solutions are crafted to optimize efficiency and deliver a seamless customer experience. Contact LeewayHertz to transform your banking and finance operations with innovative, scalable, and customized AI solutions that drive growth in today’s digital era.
How to build a financial assistance app?
While we discussed the high-level steps in implementing AI solutions for finance business in the previous section, this section delves deep into the steps required to build financial assistance applications. So, let’s start with the core understanding of financial assistance applications.
What are AI-powered financial assistance apps?
AI-powered financial assistance apps leverage artificial intelligence technologies to provide users with personalized and intelligent support in managing their finances. These applications use machine learning algorithms, natural language processing, and data analytics to offer budgeting, expense tracking, investment advice, and financial planning features. These apps aim to enhance user decision-making, optimize financial strategies, and improve overall financial well-being by analyzing user data and financial patterns.
For example, personal financial assistant apps are powerful tools designed to help users intelligently manage their finances. These applications go beyond basic budgeting and offer a wide range of functionalities, from monitoring expenses and income to providing advice on optimal investment opportunities. Some advanced features even include managing subscriptions and negotiating better rates for bills.
These personal financial assistants can exist as standalone applications or seamlessly integrate with personal banking accounts. In the latter case, users gain enhanced control and management capabilities as the software automatically retrieves transaction history and other relevant financial data. Many personal finance applications amalgamate various features to deliver a holistic user experience, offering comprehensive financial management services. The choice of features for your application should align with your business goals and cater to the needs of potential users.
Steps to build a financial assistance app
Building a financial assistance app with AI capabilities systematically integrates technology, data, and user experience. For this section we will explore an app used for intelligent investment guidance. Below are key steps to guide the development of an effective AI-driven financial app:
Define objectives and persona development
Clearly outline the goals and features of your AI-powered financial assistance app. Identify the specific financial challenges your app aims to address, such as budgeting, investment guidance, or debt management. Understanding the objectives will guide the overall design and functionality of your app. Typical applications of AI-powered financial assistant apps include but are not limited to:
- Provide users with a one-stop solution for comprehensive financial management.
- Offer personalized investment recommendations based on user financial profiles.
- Utilize AI for tailored financial advice based on user behavior and financial goals.
- Assist users in effectively managing and reducing their debts.
- Empower users with financial literacy and insights.
Intelligent investment guidance app offers personalized investment recommendations based on user financial profiles. Key features supported by this financial assistance app include providing investment insights, risk analysis, and portfolio optimization.
User research and persona development
Conduct thorough user research to understand your target audience’s needs, preferences, and pain points. Create user personas to represent different segments of your user base. This will help tailor the app to meet the diverse requirements of your users. User research is foundational to gaining insights into your target audience’s behaviors, expectations, and challenges. In the context of an AI financial app for intelligent investment guidance, the following aspects should be explored:
- Investment behavior analysis: Understand how users currently approach investment decisions, whether they are beginners seeking guidance or experienced investors looking for advanced strategies.
- Financial literacy levels: Assess potential users’ financial knowledge and literacy levels to tailor educational content accordingly.
- Technology adoption: Explore users’ comfort levels with technology, including their familiarity with AI-driven financial tools.
- Risk appetite: Determine users’ risk tolerance and appetite for various investment strategies.
- App usage patterns: Analyze how users currently interact with financial apps, including their preferences for features, interface design, and engagement frequency.
- User feedback mechanism: Establish a feedback loop to collect users’ insights regarding their app experience.
By integrating user research and persona development, the AI financial app can be crafted to align with different user segments’ specific needs and preferences. This user-centric approach enhances engagement, fosters trust, and contributes to the app’s overall success in delivering intelligent investment guidance.
Data collection and integration
Data collection and integration are foundational to building a financial investment app empowered by AI. This process involves gathering relevant financial data, ensuring compliance with privacy regulations, and implementing secure storage practices.
Gather relevant financial data:
- Transaction history: Collect comprehensive data on users’ past financial transactions to understand spending patterns and behaviors.
- Income details: Capture information about users’ income sources, enabling the app to provide insights into available funds for investment.
- Investment portfolios: Access and aggregate data related to users’ current investment portfolios, including asset allocation and performance.
- APIs integration: Integrate APIs from financial data providers (e.g., Alpha Vantage, Yahoo Finance)
Data preprocessing and cleaning
Ensure compliance with data privacy regulations and implement secure data storage practices.
Integrate APIs or other methods to collect real-time financial data, laying the foundation for robust analytics and insights.
Handle data quality
- Address missing data, outliers, and noise.
- Normalize or standardize features.
Feature extraction and selection
- Extract relevant features from the data (e.g., price-to-earnings ratio, volatility).
- Select features that impact investment decisions.
Choose AI models and algorithms
Select AI models and algorithms based on the app’s objectives, such as machine learning for predictive analysis, natural language processing for user interactions, and recommendation systems for personalized advice. Choose appropriate models:
- Regression models: Predict stock prices or portfolio returns.
- Classification models: Recognize buy/sell signals.
- Time series models: Forecast future prices.
Model training and validation
- Split data: Divide data into training and validation sets.
- Train ML models: Train machine learning models using historical data.
- Validate model performance: Validate the performance of the trained models using metrics such as RMSE (Root Mean Square Error) for regression tasks or accuracy for classification tasks.
Develop user-friendly interface
Create intuitive and user-friendly interfaces for both web and mobile platforms. Design interactive dashboards and visualizations to present financial insights and recommendations in an understandable manner. Prioritize a seamless user experience to encourage engagement and adoption. Building user-friendly app interfaces involves:
Understanding user needs
- Conduct research to identify user preferences and expectations.
- Define key features based on user priorities.
Responsive design
- Implement responsive design for seamless access across various devices.
- Prioritize mobile responsiveness for widespread accessibility.
Visualizing financial insights
- Create interactive dashboards with charts and graphs for an at-a-glance view.
- Utilize visualizations to present complex financial insights comprehensibly.
Intuitive navigation
- Design intuitive user flows and clear navigation paths.
- Ensure easy access to essential features with minimal steps.
Usability testing and feedback
- Conduct usability testing to identify pain points and areas for improvement.
- Incorporate user feedback for iterative enhancements to the interface.
Portfolio optimization and diversification
Diversification is a fundamental principle in portfolio optimization that involves spreading investments across different asset classes to reduce risk. Optimization techniques, such as the Markowitz portfolio theory, help in creating diversified portfolios that balance risk and return based on investor preferences.
- Markowitz portfolio theory
- Developed by Harry Markowitz, this theory emphasizes the importance of diversification in optimizing investment portfolios.
- It mathematically determines the optimal allocation of assets to achieve the maximum expected return for a given level of risk (volatility).
- Balancing risk and return
- Investors have varying risk tolerance levels and return expectations. Portfolio optimization aims to strike a balance by adjusting the mix of assets to align with an investor’s risk appetite while maximizing potential returns.
Risk assessment and management
Calculate risk metrics
- Compute risk measures (e.g., Sharpe ratio, Value at Risk).
- Implement risk management strategies (e.g., stop-loss orders).
Sentiment analysis and news integration
Monitor news: Sentiment analysis and real-time news integration are crucial in staying informed about market developments.
- Sentiment analysis using NLP
- Natural Language Processing (NLP) techniques analyze news articles and social media to gauge sentiment.
- NLP helps determine whether news sentiment is positive, negative, or neutral, providing additional context for investment decisions.
- Real-time news feeds
- Integrating real-time news feeds into the app ensures that users have access to the latest information.
- News about market trends, economic indicators, or geopolitical events can influence investment decisions.
Test and iterate
Conduct thorough testing to identify and rectify bugs, glitches, and potential security vulnerabilities. Gather user feedback during beta testing to make iterative improvements and enhance the overall user experience. Rigorous testing ensures the app’s reliability and performance.
Implement security measures
Prioritize the security of user data and financial information. Utilize encryption protocols and secure authentication methods, and follow industry best practices to safeguard sensitive data from potential threats. Establish trust with users by ensuring the highest standards of data protection.
Deployment and monitoring
Deploy the app to the intended platforms and monitor its performance in real-world scenarios. Implement analytics tools to track user engagement, app usage patterns, and the effectiveness of AI-driven features. Use monitoring data to make informed decisions for future updates and optimizations.
Continuous learning and improvement
Implement mechanisms for continuous learning and improvement of AI models. Regularly update algorithms based on user interactions, changing financial trends, and emerging technologies to ensure the app remains relevant and effective. Leverage user feedback and analytics for iterative enhancements.
Provide ongoing support
Offer continuous customer support to address user inquiries, troubleshoot issues, and gather insights for future enhancements. Regularly update the app to introduce new features and improvements, ensuring its long-term value for users.
- Provide educational content on investment strategies.
- Stay agile and responsive to evolving user needs and technological advancements.
- Offer customer support for app users.
Conversational AI in the banking and financial services industry
Conversational AI in the banking and financial services industry encompasses technologies such as chatbots and virtual agents that enable users to engage in conversations. These technologies leverage extensive data, machine learning, and natural language processing to mimic human interactions. They can understand both spoken and written inputs, decipher the meanings and communicate in multiple languages. This technology enables banks and financial institutions to provide a more personalized and convenient customer experience, streamline various banking processes, and enhance customer engagement across multiple channels, including websites, mobile apps, messaging platforms, and even voice-activated devices.
Conversational AI offers a significant advantage by providing 24/7 assistance. Whether it’s answering questions about account balances, helping with money transfers, or offering financial advice, these AI-powered systems are ready to assist instantly without customers having to wait in lines or worry about business hours. This ensures a more sensible and hassle-free experience for customers and fosters greater customer satisfaction and loyalty.
A conversational AI chatbot relies on five essential components that work together to enable computers to engage in human-like conversations. These components include:
- Natural Language Processing (NLP): It empowers computer systems to grasp and respond to human language in a manner that feels intuitive to customers. It includes understanding word meanings and sentence structures and the ability to adapt to idiomatic expressions and colloquialisms commonly used in banking conversations.
- Machine Learning (ML): ML enables computers to acquire knowledge and glean insights from data without requiring explicit, manual programming. Machine learning algorithms continuously refine themselves as they encounter more data. Within the context of conversational AI in the banking sector, machine learning plays a pivotal role in training computers to recognize patterns of financial data and construct models that simulate various banking processes, sometimes even emulating human thought processes.
- Text analysis: Text analysis involves extracting meaningful information from textual data, breaking down sentences into their constituent parts (e.g., subjects, verbs, objects), and categorizing words (e.g., nouns, verbs, adjectives). This practice helps decipher sentence meanings and relationships between words and discern sentiment, whether it’s positive or negative.
- Computer vision: Computers are endowed with the capability to interpret and make sense of digital images. This involves identifying objects present in images, ascertaining their locations and orientations, and understanding the overall context of these images by analyzing their contents and the interrelationships between various objects in the image. Moreover, computer vision extends its capabilities to detect emotional cues conveyed through people’s expressions in images.
- Speech recognition: It empowers computers to comprehend human speech by accomplishing several critical tasks. This includes identifying distinct sounds within spoken sentences, understanding the underlying grammatical structures and syntax, and converting spoken words into written text. Furthermore, it plays a significant role in deciphering word meanings, deducing emotions from spoken expressions, and capturing the broader context of spoken conversations.
Benefits of AI in the banking and finance industry
AI is reshaping the banking and financial services industry by providing numerous benefits that help banks and financial institutions improve operations, enhance customer experiences, and increase profitability. Here are some of the key benefits of AI in banking and financial services:
Improved decision-making: AI’s real-time data analysis empowers banks to make informed decisions, optimizing investment strategies and credit risk assessments. This reduces default risks, enhances profitability, and uncovers new opportunities. By harnessing AI’s analytical capabilities, financial institutions gain a competitive edge, ensuring prudent lending decisions and effective risk management in today’s dynamic financial landscape.
Enhanced customer experiences: AI-driven chatbots and virtual assistants offer personalized and round-the-clock customer support, ensuring that customers receive assistance when needed, without time constraints. This accessibility and responsiveness significantly enhance the customer experience, as customers can get immediate help with their inquiries or issues. Consequently, improved customer satisfaction is achieved due to quicker query resolution and convenience, leading to increased customer loyalty as customers tend to stick with businesses that provide efficient and tailored support, fostering long-term relationships and potentially driving additional business.
Increased efficiency: AI automation streamlines labor-intensive tasks like compliance monitoring, loan underwriting, and fraud detection, significantly reducing time and operational expenses. This boosts efficiency as AI systems work tirelessly and swiftly to handle large volumes of data and make consistent decisions. The reduced reliance on manual efforts also frees up skilled personnel to dedicate their expertise to more strategic and value-added activities, ultimately improving the organization’s productivity and ability to serve customers effectively.
Enhanced security: AI plays a crucial role in bolstering data security for banks through the implementation of robust encryption techniques. Around 33 percent of AI applications worldwide are focused on enhancing security for financial institutions. These advanced encryption methods transform sensitive customer data into complex, unreadable code, effectively shielding it from unauthorized access. By continuously adapting encryption protocols based on evolving threats, AI ensures that even the most determined cybercriminals face formidable barriers when attempting to breach the bank’s defenses. This proactive approach to data protection is essential in maintaining customer trust and safeguarding critical financial information.
Improved risk management: AI algorithms synthesize data from diverse sources like customer behavior, market trends, and economic indicators. This multifaceted analysis enables the early detection of potential risks, allowing banks and financial institutions to take preemptive actions. As a result, risk management is significantly enhanced, reducing the likelihood of defaults and preserving financial institutions’ investments. This proactive stance safeguards their assets and fosters a more resilient and secure financial landscape.
Reduced cost: AI-powered recommendations and targeted marketing campaigns enable banks to engage with customers more effectively. By tailoring services and offers to individual preferences, banks can increase customer loyalty and reduce the costs associated with acquiring new customers. Additionally, AI-driven chatbots and virtual assistants streamline customer interactions, efficiently handling routine inquiries and transactions. This reduces the reliance on human customer support agents and tellers, resulting in lower labor costs and improved operational efficiency.
Reduced bias: AI indeed offers a powerful solution to address bias in credit scoring in banks and financial institutions. By leveraging AI algorithms to analyze customer data, lending decisions can be made based solely on financial factors, mitigating the influence of potentially discriminatory variables like race, gender, or age. This ensures that the credit scoring process is fair and unbiased, promoting equal access to financial services for all individuals. Moreover, AI-driven systems continuously learn and adapt, reducing the risk of perpetuating historical biases and fostering a more inclusive and equitable lending environment. AI helps banking and financial institutions align their credit evaluation processes with fairness and equal opportunity principles.
Automated workflows: AI-powered automation enables the streamlining of workflows and processes, enabling autonomous and responsible operations while enhancing decision-making and service delivery. In banking, AI-driven automation is projected to save nearly $70 billion by 2025 by automating routine tasks such as accounts payable, financial closing processes, and customer service operations.
Accuracy and speed: AI significantly enhances accuracy and speed in financial services by reducing manual errors in data processing, analytics, document processing, onboarding, customer interactions, and other tasks through automation and consistent algorithmic processes. AI processes vast amounts of information much faster than humans can, enabling the discovery of patterns and relationships in data that may be overlooked by humans. This rapid analysis provides quicker insights for decision-making, risk modeling, compliance management, and various other aspects of financial operations.
Availability: Financial institutions leverage AI to provide personalized customer assistance, address individual needs, and efficiently manage customer finances. When deployed in the cloud, AI and machine learning (ML) systems operate continuously, handling assigned activities seamlessly and without interruption. This ensures consistent and accessible support for customers, ultimately enhancing their overall experience with financial services.
Maximizing efficiency in finance and banking: The impact of AI agents
An AI agent is an artificial intelligence system designed to operate autonomously towards achieving specific objectives. In contrast to traditional automation methods that rely on human-defined triggers and actions, AI agents leverage advanced algorithms and large language models like Gemini or GPT-4 to interpret goals, generate task lists, gather information from the internet, make decisions, and complete tasks autonomously. These intelligent AI agents, developed using advanced tools like AutoGen Studio and CrewAI, mimic human cognitive processes by continuously iterating, gathering feedback, and adapting their strategies until the desired outcome is achieved.
What are the key applications of AI agents in enhancing finance and banking operations?
AI agents play a significant role in the finance and banking sector by offering a range of capabilities that enhance efficiency, accuracy, and customer experience. Here’s how AI agents help in this domain:
- Fraud detection and prevention: AI agents can analyze vast amounts of transactional data in real time to identify suspicious patterns or anomalies that may indicate fraudulent activity. They can help financial institutions detect and prevent fraudulent transactions more effectively than traditional methods.
- Risk management: AI agents utilize advanced algorithms to assess and manage financial risk. They can analyze market trends, historical data, and other relevant factors to provide insights that help financial institutions make informed decisions about investments, loans, and other financial products.
- Credit scoring and lending decisions: AI agents leverage machine learning algorithms to analyze customer data and assess creditworthiness more accurately. They can automate the credit scoring process, streamline loan approvals, and offer personalized lending solutions based on individual risk profiles.
- Algorithmic trading: AI agents are employed in algorithmic trading systems to execute high-frequency trades at optimal times based on market conditions and predefined strategies. These agents can analyze large datasets and react swiftly to changes in the market, potentially generating profits for financial institutions.
- Portfolio management: AI agents assist in portfolio management by analyzing investment goals, risk tolerance, and market trends to recommend asset allocations and investment strategies tailored to individual investors’ needs.
- Compliance and regulatory assistance: AI agents help financial institutions stay compliant with regulatory requirements by monitoring transactions, identifying potential compliance issues, and flagging suspicious activities that may violate regulations.
- Personalized financial services: AI agents analyze customer data to offer personalized financial services and product recommendations, such as investment opportunities, insurance plans, and savings strategies, based on individual financial goals and preferences.
Overall, AI agents in the finance and banking sector contribute to improved operational efficiency, better risk management, enhanced customer service, and more informed decision-making, ultimately benefiting both financial institutions and their customers.
Empower your finance and banking operations with LeewayHertz’s AI agent development services
If you’re looking to empower your finance and banking operations with advanced AI agent development services, LeewayHertz is your ideal partner. Specializing in crafting custom AI agents for diverse industries, including finance and banking, we streamline financial operations, enhance fraud detection, and redefine customer interactions. Benefit from expert consultation and strategy formulation tailored to AI agent deployment, ensuring seamless integration and ongoing optimization. Our custom AI agent development empowers businesses with versatile and adaptive solutions, leveraging state-of-the-art technology such as Llama 2, PaLM 2, and GPT-4. Trust in our expertise to deliver AI agents that automate tasks, drive efficiency, and propel your business into the future.
Challenges and considerations in using AI for finance
1. Data quality
Challenge: The quality and reliability of data used to train AI models significantly impact the accuracy and effectiveness of financial applications. Financial data can be vast, complex, and subject to inherent biases and inaccuracies, which can compromise the performance of AI algorithms.
Considerations:
- Ensure data accuracy and integrity through robust data governance practices.
- Implement data cleansing and normalization techniques to mitigate biases and inconsistencies.
- Invest resources in data labeling, verification, and validation to enhance data quality for AI applications.
- Collaborate with data providers and regulators to access high-quality, compliant datasets.
2. Black-box effect
Challenge: AI models often operate as “black boxes,” meaning they make decisions without providing clear explanations or justifications. This lack of transparency poses challenges for interpreting results and understanding the underlying reasoning behind AI-driven decisions.
Considerations:
- Employ explainable AI (XAI) techniques to enhance the transparency and interpretability of AI models.
- Use model-agnostic interpretability methods to analyze and visualize AI model behaviors.
- Facilitate human-AI collaboration to combine machine-generated insights with human expertise.
- Educate stakeholders and regulators about AI model transparency and its implications for decision-making.
3. Narrow focus
Challenge: AI algorithms are typically designed to solve specific tasks and may struggle to generalize or adapt to new scenarios beyond their training data. This limitation can hinder the scalability and versatility of AI applications in finance.
Considerations:
- Define clear objectives and use cases for AI implementation to align with specific business needs.
- Continuously evaluate and adapt AI models to evolving market conditions and regulatory requirements.
- Explore ensemble learning and transfer learning techniques to improve AI model generalization.
- Leverage hybrid AI approaches that combine specialized algorithms with human judgment for complex decision-making.
4. Privacy and security
Challenge: Financial data is highly sensitive and subject to stringent privacy regulations. AI applications must comply with data protection laws (e.g., GDPR, CCPA) to safeguard customer information and mitigate cybersecurity risks.
Considerations:
- Implement robust data encryption and access controls to protect sensitive financial data.
- Conduct regular security audits and vulnerability assessments to identify and address potential threats.
- Adhere to regulatory frameworks and industry standards for data privacy and cybersecurity.
- Foster a culture of data ethics and accountability to ensure the responsible use of AI technologies.
5. Bias and fairness
Challenge: AI models can inherit biases from training data, leading to discriminatory outcomes in financial decision-making. Ensuring fairness and mitigating bias is essential for maintaining trust and compliance in AI-driven applications.
Considerations:
- Conduct bias detection and fairness assessments to identify and address algorithmic biases.
- Implement algorithmic transparency and fairness testing throughout the AI lifecycle.
- Utilize diverse and representative datasets to reduce bias and promote inclusivity in AI applications.
- Educate AI developers and stakeholders about ethical considerations and best practices in bias mitigation.
6. Ethical issues
Challenge: AI systems can raise ethical concerns related to accountability, transparency, and human oversight in automated decision-making processes. Addressing ethical considerations is crucial for building trust and credibility in AI-driven finance.
Considerations:
- Establish ethical guidelines and governance frameworks for responsible AI deployment.
- Promote transparency and explainability of AI algorithms to enhance trust and accountability.
- Enable human oversight and intervention in critical decision-making processes involving AI.
- Foster collaboration between interdisciplinary teams to address ethical dilemmas and societal impacts of AI in finance.
7. Regulation
Challenge: The regulatory landscape for AI in finance is evolving, with new laws and guidelines shaping the deployment and use of AI technologies. Financial institutions must navigate complex regulatory requirements while ensuring compliance and risk management.
Considerations:
- Stay informed about evolving regulations (e.g., AML, KYC, GDPR) governing AI applications in finance.
- Collaborate with policymakers and regulators to advocate for responsible AI governance and compliance.
- Implement audit trails and documentation processes to demonstrate regulatory compliance in AI operations.
- Invest in regulatory compliance training and resources for AI practitioners and decision-makers.
Future of AI in the banking and finance industry
The future of AI in banking and financial services is an exciting prospect that promises to reshape the industry in many ways. With the rapid advances in AI technology and the increasing availability of data, banks and financial institutions are uniquely positioned to harness AI’s power to drive business growth, improve customer experiences, and reduce risks.
One of AI’s most significant ways to redefine operations in the banking industry is through enhanced customer experiences. AI-powered chatbots and virtual assistants can provide customers with personalized financial advice and support, offering previously impossible convenience. These virtual assistants can help customers with various tasks like account inquiries, money transfers, and investment recommendations. Moreover, AI can optimize customer journeys by analyzing customer behavior, preferences, and feedback and creating personalized experiences that better meet their needs.
In addition to improving customer experiences, AI is expected to play a significant role in risk management in the banking and financial services industry. By analyzing vast amounts of data in real-time, machine learning algorithms can detect and prevent fraudulent activities, reduce the risk of errors, and enhance overall security. AI can also be used to analyze customer data and identify potential risks, such as customers who are at risk of defaulting on loans or credit cards, enabling banks and financial institutions to take proactive measures to mitigate these risks.
Furthermore, AI is expected to improve efficiency and reduce costs for banks and financial institutions. By automating manual processes, such as document underwriting and compliance checks, banks can process applications more quickly and efficiently, reducing the time and costs of manual processes. AI can also streamline operations, optimize workflows, and reduce the need for human intervention, freeing up resources for other critical tasks. Another example of the potential of AI in the banking industry is its application in loan underwriting. By analyzing customer data and automating credit checks and approvals, banks can reduce the time and costs associated with manual processes and process loans more quickly and efficiently. Furthermore, AI can analyze customer data and identify potential opportunities for cross-selling and upselling, allowing banks and financial institutions to offer additional products and services that better meet the needs of their customers. The potential applications of AI in banking and financial services are almost limitless, and the industry is only beginning to scratch the surface of what is possible.
Conclusion
Integrating AI in the banking industry has brought remarkable advancements and possibilities. AI’s presence in banking has significantly enhanced operational efficiency, risk management, customer experiences, and decision-making processes. AI-powered technologies, such as machine learning algorithms and data analytics, have enabled banks to analyze vast amounts of data in real time, identifying patterns, trends, and anomalies that help mitigate risks and make more informed decisions. Furthermore, AI-driven chatbots and virtual assistants improve customer interactions by providing personalized assistance, addressing queries promptly, and streamlining routine transactions. The application of AI in credit scoring has improved accuracy and speed, allowing banks to make informed lending decisions and expand access to financial services. Additionally, AI’s contribution to fraud detection and prevention has been instrumental in safeguarding banks and customers from fraudulent activities. Looking ahead, the future of AI in banking holds great promise. Continued advancements in AI technology, coupled with ongoing collaborations between financial institutions and AI experts, will drive further innovation and enable banks to deliver enhanced services, improve efficiency, and stay competitive in an ever-evolving digital landscape.
Redefine your financial services with AI development solutions tailored for the Banking and Finance industry. Contact our expert team of AI developers to learn about AI-related consultancy and development services.
Start a conversation by filling the form
All information will be kept confidential.
FAQs
How is AI impacting the banking and finance industry?
AI transforms banking and finance through process automation, elevated decision-making, enriched customer interactions, cost reduction and more. One prominent way it helps businesses in this field is by enabling data analysis, making it easy for them to make data-driven decisions. Additionally, AI excels in fraud detection, safeguarding against unauthorized activities while enhancing risk management practices. The personalized touch of AI-driven solutions fosters tailored customer experiences, reshaping the landscape in this industry.
What is conversational AI, and how is it used in banking?
Conversational AI, driven by natural language processing and machine learning capabilities, emulates human interactions. This technology is used to offer round-the-clock support, seamlessly addressing customer inquiries and enhancing engagement across multiple channels, including websites, mobile apps, and messaging platforms. Through chatbots and virtual assistants, financial institutions provide instant assistance, contributing to a more accessible and responsive customer experience in the digital landscape.
Can AI help in personalized financial planning?
Yes, AI plays a crucial role in personalized financial planning by analyzing individual financial data, understanding goals, assessing risk tolerance, and recommending tailored investment strategies. It provides customized asset allocation strategies based on customer profiles. Utilizing this comprehensive understanding, AI engages in a dialogue with customers to establish clear financial objectives. With the ability to adapt and learn, AI formulates personalized asset allocation strategies to optimize each customer’s unique financial position, ensuring a tailored and informed approach to wealth management.
How does AI contribute to fraud detection in banking?
AI algorithms play a pivotal role in bolstering security within the financial sector by processing extensive datasets in real-time. These algorithms swiftly identify irregularities and potential fraud indicators through advanced pattern recognition. This proactive approach enables the timely detection of suspicious transactions, effectively preventing financial losses. By providing an additional layer of defense, AI enhances overall security, safeguarding the interests of customers and financial institutions in the rapidly evolving landscape of digital transactions.
What benefits does AI bring to the banking and finance industry?
In the finance industry, including banking, AI transforms operations by optimizing decision-making, elevating customer experiences, boosting efficiency, and fortifying security. This technology reduces costs through streamlined processes and ensures a competitive edge in the dynamic digital landscape. Its multifaceted impact extends from personalized customer interactions to proactive risk management, marking a paradigm shift in the financial industry.
How does AI contribute to improving the overall security of financial transactions?
AI plays a crucial role in transaction security through real-time pattern recognition and anomaly detection. By meticulously scrutinizing transaction data, it swiftly identifies potential fraudulent activities, enabling proactive intervention. This heightened vigilance enhances overall security, preventing unauthorized transactions and safeguarding customer funds. The precision of AI-driven systems in identifying irregular patterns contributes significantly to minimizing financial risks and ensuring a secure transaction environment in the banking and finance industry.
How does LeewayHertz utilize AI to enhance banking and finance operations?
LeewayHertz leverages AI technologies to streamline banking and finance operations by automating fraud detection, risk management, and customer service processes. Our solutions enhance efficiency, reduce manual effort, and provide real-time insights for better decision-making.
Can LeewayHertz's AI solutions be customized for specific banking needs?
Yes, LeewayHertz specializes in developing tailored AI solutions for banking and finance institutions. Our experts work closely with clients to understand their unique requirements, offering customized AI applications that meet their specific needs, from personalized customer experiences to advanced data analytics.
What are AI-powered financial assistance apps, and how do they work?
AI-powered financial assistance apps leverage artificial intelligence and machine learning to offer intelligent financial services. They analyze user data, provide personalized insights, and automate tasks such as budgeting, investment guidance, and expense tracking. These apps adapt to user behaviors and market changes through continuous learning, delivering more tailored and effective financial assistance.
How do AI-powered financial assistance apps benefit users?
AI-powered financial assistance apps provide several advantages. They offer personalized financial insights, help optimize investment portfolios, and streamline budget management. By automating routine tasks and analyzing data, these apps save time, reduce manual errors, and contribute to more informed financial decision-making. Additionally, the adaptability of AI models ensures that recommendations stay relevant to the user’s financial goals and preferences.