AI in store replenishment: Navigating the future of retail operations
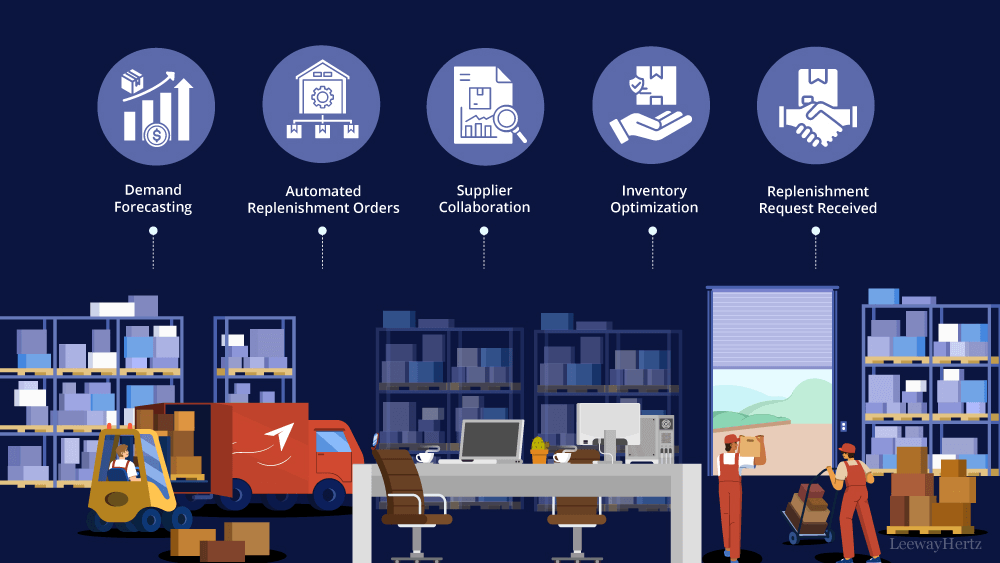
From the early days of barter systems to today’s digital marketplaces, our constant quest for technological progress has shaped the path of commerce. Today, as we have reached the era of constant digital advancements, it’s not only personal lives that are changing; entire industries are undergoing profound transformations. Among these industries, retail stands out as a prime example of technology’s disruptive impact. The adoption of advanced technologies, such as artificial intelligence, has been a pivotal force driving this change. Notably, AI in the retail market is poised for remarkable growth, with predictions indicating an expansion from USD 7.30 billion in 2023 to a substantial USD 29.45 billion by 2028, reflecting an impressive CAGR of 32.17%. One area where this transformation is particularly evident is in the store replenishment process.
Thanks to AI, stores have become adept at anticipating customer needs. AI employs ingenious methods, such as predictive algorithms that anticipate consumer preferences and real-time sales data analysis, to ensure that stores consistently stock the right products. It’s akin to having an exceptionally intelligent assistant, enabling retailers to manage inventory and swiftly adapt to evolving consumer preferences efficiently.
This article dives into the following aspects of AI in store replenishment, providing a comprehensive overview of how artificial intelligence is transforming the retail industry:
- What is store replenishment?
- Significance of store replenishment in retail operations
- Challenges associated with traditional store replenishment methods
- Applications of AI in store replenishment
- Benefits of AI in store replenishment
- How does LeewayHertz’s generative AI platform streamline store replenishment workflows?
- AI technologies used in store replenishment
- How to implement AI in store replenishment?
- Exploring the future of AI in store replenishment
What is store replenishment?
Store replenishment forms the backbone of efficient retail operations, ensuring that goods are available on the shelves at the right times and in the right quantities. This process involves regularly restocking a store’s inventory to maintain ideal levels. It’s a crucial balancing act: on the one hand, avoiding stockouts, where items are unavailable when customers seek to purchase them, and on the other, preventing overstocks, which tie up cash and consume valuable shelf space. Effective replenishment requires careful consideration of consumer demand patterns, seasonality, and supply chain dynamics to ensure that inventory levels are optimally managed.
Significance of store replenishment in retail operations
Store replenishment holds a pivotal role in the success of retail operations, shaping the overall shopping experience for customers and influencing a retailer’s bottom line. This critical process ensures that products are consistently available to meet customer demands while maintaining efficient inventory levels. The significance of store replenishment can be understood through the following key points:
- Customer satisfaction: Adequate store replenishment ensures that customers can easily find and purchase the products they desire. When shelves are well-stocked, customers are more likely to find the items they need, leading to positive shopping experiences. Conversely, stockouts can frustrate customers and drive them to seek alternatives, potentially resulting in lost sales and customer loyalty.
- Sales maximization: Optimized store replenishment directly impacts a retailer’s sales revenue. By having products readily available, retailers can capture potential sales opportunities without the risk of losing customers due to product unavailability. Effective replenishment strategies contribute to achieving higher sales targets and revenue growth.
- Inventory efficiency: Store replenishment prevents inventory imbalances. Maintaining the right balance between excess inventory and stockouts is essential to managing costs effectively. Overstocked items tie up capital and storage space, while stockouts lead to missed sales and dissatisfied customers. Balancing these two factors through efficient replenishment helps control costs and improve overall inventory turnover rates.
- Operational streamlining: Implementing systematic store replenishment processes minimizes manual intervention and ensures the optimal utilization of resources. This, in turn, frees up employees’ time to focus on providing quality customer service and other value-added tasks, enhancing the overall efficiency of store operations.
- Brand reputation: Consistently well-stocked shelves reflect positively on a retailer’s brand image. A reputation for having products available when needed builds trust and encourages customer loyalty. Conversely, a reputation for frequent stockouts can tarnish a brand’s image and drive customers away.
- Data-driven insights: Store replenishment generates valuable data on product demand, sales patterns, and customer preferences. By leveraging this data, retailers can gain insights into market trends, consumer behavior, and the effectiveness of promotional strategies. These insights inform better decision-making and strategy planning.
- Resource allocation: Effective store replenishment aligns resource allocation with demand. By replenishing items based on accurate demand forecasts, retailers can allocate resources efficiently, reducing waste and optimizing supply chain operations.
- Sustainability and waste reduction: Effective store replenishment strategies can also play a crucial role in enhancing sustainability within retail operations. By optimizing inventory levels and reducing overstock, retailers can minimize waste, especially of perishable goods. This not only has environmental benefits but also aligns with the growing consumer preference for sustainable and responsible retail practices. Additionally, efficient replenishment can lead to reduced energy consumption and lower carbon footprints, as it can decrease the frequency and volume of deliveries needed to restock stores. Incorporating this aspect of sustainability and waste reduction adds another dimension to the importance of store replenishment in modern retail.
In essence, store replenishment is the backbone of a successful retail operation. As customer expectations evolve and competition intensifies, implementing advanced technologies like Artificial Intelligence in store replenishment can unlock new levels of efficiency, accuracy, and responsiveness, ensuring retailers remain at the forefront of their industry.
Launch your project with LeewayHertz!
Leverage AI tools for store replenishment and enhance your retail or e-commerce business. Reach out to us now to explore tailor-made solutions that align seamlessly with the unique needs of your business.
Challenges associated with traditional store replenishment methods
While traditional and manual store replenishment methods have been the norm for years, they come with a set of challenges that can hinder efficiency, accuracy, and customer satisfaction. These challenges underscore the need for modernizing replenishment processes through advanced technologies like Artificial Intelligence. Here are some of the key challenges associated with traditional/manual store replenishment:
- Limited data utilization: Traditional methods often rely on historical sales data and basic demand forecasts. This limited scope may overlook important factors such as seasonality, emerging consumer trends, and external events that impact product demand. Consequently, replenishment decisions may not accurately reflect the dynamic nature of the market.
- Human error: Manual calculations and decision-making are prone to human error. Mistakes in determining to reorder quantities, lead times, and safety stock levels can lead to understocking or overstocking situations. These errors not only impact sales but also tie up capital in excess inventory or result in missed sales opportunities.
- Lack of real-time insights: Manual processes struggle to provide real-time insights into inventory levels, product movement, and customer behavior. Without up-to-date information, retailers may miss opportunities to make timely adjustments to their replenishment strategies.
- Inefficient resource allocation: Traditional methods may not effectively allocate resources based on demand. Overreliance on manual calculations can lead to suboptimal distribution of products across stores, resulting in excess inventory in some locations and stockouts in others.
- Difficulty in scaling: As retail operations expand, manual replenishment becomes increasingly complex to manage across multiple locations. Coordinating replenishment efforts becomes challenging, and inconsistencies in execution may arise.
- Reactive responses: Manual processes often result in reactive responses to stockouts or excessive inventory. Retailers may rely on expedited orders to address stockouts, which incurs higher costs, while surplus inventory might lead to markdowns or losses.
Applications of AI in store replenishment
AI technologies and techniques can enhance various aspects of the replenishment process, leading to improved efficiency, accuracy, and cost savings. Here are some key roles that AI plays in the store replenishment process:
Demand forecasting
Demand forecasting with AI involves utilizing advanced machine learning algorithms to examine past sales data and spot patterns, account for seasonality and trends, and take into account external factors like holidays or promotional events. The integration and analysis of this wide range of data points enable AI models to produce extremely precise forecasts of future client demand. This allows merchants to proactively change their inventory levels, optimize replenishment orders, and guarantee that items are available when and where they are required, increasing consumer satisfaction, lowering stockout rates, and cutting expenses associated with excess inventory.
Reorder point calculation
AI algorithms play a crucial role in establishing the ideal reorder point for each product by factoring in variables such as the time it takes to restock (lead time), the degree to which demand fluctuates (demand variability), and the desired level of service to customers. This enables the system to dynamically calculate the point at which an order should be initiated, ensuring that replenishment orders are triggered precisely when needed to prevent stockouts. By aligning these factors, businesses can maintain optimal inventory levels that accommodate demand fluctuations, minimizing the risk of unmet customer needs while also avoiding the excess costs associated with carrying surplus inventory.
Dynamic pricing
AI’s capacity to analyze up-to-the-minute market dynamics, competitor pricing strategies, and evolving demand trends empowers retailers to implement dynamic pricing approaches. By considering these multifaceted factors, AI can provide timely recommendations for adjusting prices in response to changing circumstances, such as increased demand or competitive shifts. This enables retailers to optimize profitability by capturing the highest possible value for their products while also encouraging efficient stock movement. Such dynamic pricing strategies ensure that products remain competitively priced, minimizing the risk of overstock due to unreasonably high prices and mitigating stockouts by enticing customers with attractive offers during periods of heightened demand.
Supplier collaboration
AI in store replenishment fosters enhanced supplier collaboration by facilitating more transparent and data-driven relationships. Through AI-driven demand forecasting and inventory management, retailers can provide suppliers with accurate, real-time information about product demand and stock levels. This enables suppliers to optimize production and delivery schedules, minimizing overproduction or supply chain disruptions. Additionally, AI can automate communication between retailers and suppliers, streamlining the ordering and restocking process. The result is a more efficient and collaborative supply chain ecosystem where both retailers and suppliers benefit from reduced costs, improved product availability, and better overall performance.
Automated replenishment orders
AI-driven systems transform the replenishment process by automating the creation and transmission of replenishment orders to suppliers. By leveraging historical sales data, current inventory levels, and sophisticated algorithms, these systems can autonomously determine when to trigger orders for restocking. This automation minimizes the reliance on manual intervention, reducing the chances of human error and freeing up valuable personnel resources. Furthermore, the streamlined automation accelerates the ordering cycle, ensuring that replenishment requests are dispatched promptly. Consequently, retailers can maintain optimal inventory levels while expediting the overall replenishment process, leading to enhanced efficiency, reduced administrative burden, and a more responsive supply chain.
Launch your project with LeewayHertz!
Leverage AI tools for store replenishment and enhance your retail or e-commerce business. Reach out to us now to explore tailor-made solutions that align seamlessly with the unique needs of your business.
Inventory optimization
AI-based inventory optimization utilizes advanced algorithms to meticulously analyze a range of critical factors, thereby achieving a balance between minimizing stockouts and reducing overstock. These factors include lead times (the period required to replenish stock), product shelf life (especially important for perishable items), historical sales trends, customer preferences, and regional demand variations. Machine learning algorithms play a key role, dynamically processing these diverse variables to predict future inventory needs accurately. They employ predictive analytics, drawing on historical data and market trends to forecast demand, enabling businesses to adjust inventory levels proactively. This real-time data analysis facilitates immediate responses to sudden market shifts or supply chain disruptions. Consequently, AI’s precise stock level recommendations help avoid stockouts, ensuring product availability for customers, and prevent overstock situations, which can tie up capital, consume storage space, and lead to potential waste due to products expiring or becoming obsolete. The result is a more efficient, responsive inventory management system, significantly enhancing profitability, customer satisfaction, and overall resource utilization in the supply chain.
Data integration
The capacity of AI to combine data from several sources, including inventory databases, point-of-sale systems, and outside market data, results in a thorough knowledge of replenishment needs. Businesses may learn more about consumer purchase habits, current stock levels, and market trends thanks to this information synthesis. Analyzing this combined data, AI can produce useful recommendations for optimizing inventory levels and replenishment orders. As a result, stock management becomes more precise and responsive, reducing the possibility of stockouts and overstock conditions and eventually improving the replenishment process’ overall effectiveness.
Adaptive learning
AI algorithms exhibit a remarkable capacity to evolve alongside shifting market dynamics and evolving consumer preferences. As they process and analyze new data over time, these algorithms continuously refine their understanding of demand patterns, inventory movements, and customer behaviors. This adaptability enables AI to make increasingly precise replenishment recommendations, effectively mitigating the impact of changing circumstances, such as seasonal fluctuations or unexpected shifts in consumer demand. By learning from the most recent data, AI-driven replenishment systems enhance their accuracy and responsiveness, ensuring that inventory levels are optimally aligned with real-time customer needs.
Shelf space optimization and product placement
AI can significantly enhance how retailers manage shelf space and product placement within their stores. By analyzing customer traffic patterns, purchase history, and product interaction data, AI algorithms can identify the most effective layout for products, maximizing visibility and accessibility. This optimization leads to improved customer experiences and potentially increased sales. AI can also recommend adjustments to shelf space allocation based on changing demand patterns, ensuring that high-demand products are given priority placement and sufficient space. This level of dynamic optimization is particularly crucial in retail environments where space is a premium and customer engagement with products is key to driving sales.
Multi-channel replenishment optimization
As omnichannel retail continues to gain prominence, AI emerges as a crucial tool for enhancing replenishment strategies across diverse sales channels, encompassing brick-and-mortar stores, e-commerce platforms, and mobile apps. Through sophisticated algorithms, AI synchronizes inventory levels and streamlines fulfillment processes, facilitating a cohesive and uninterrupted shopping journey for customers, irrespective of the channel they opt for. This seamless integration improves operational efficiency and enhances customer satisfaction by offering consistent product availability across all touchpoints, thereby reinforcing brand loyalty and driving revenue growth.
Promotion optimization
AI plays a pivotal role in analyzing the influence of promotions and discounts on sales patterns. It offers a nuanced understanding that helps refine replenishment strategies. AI conducts meticulous analysis, evaluating factors such as promotion effectiveness, timing, and duration. This helps retailers adjust inventory levels to meet increased demand during promotions while avoiding excess stock. This adaptive approach not only ensures that shelves remain adequately stocked during peak periods but also minimizes the likelihood of inventory excess post-promotion, optimizing resource allocation and maximizing profitability. By leveraging AI-driven insights, retailers can navigate promotional dynamics with precision, fostering agility in inventory management and enhancing overall operational efficiency.
Customer behavior analysis
AI can analyze customer behavior data collected through various channels, such as loyalty programs, mobile apps, and POS systems, to understand purchasing patterns and preferences. Employing machine learning algorithms, it adeptly segments customers according to their buying habits, enabling tailored replenishment strategies. For instance, AI identifies high-value customers exhibiting consistent purchases of specific items and directs efforts towards prioritizing replenishment of these products in stores frequented by such clientele. This personalized approach fosters heightened sales and cultivates elevated levels of customer satisfaction, fortifying brand loyalty and propelling overall business growth.
Assortment planning
AI transforms assortment planning by personalizing product selections at individual store levels through the meticulous analysis of comprehensive customer data. By leveraging advanced algorithms, AI empowers retailers to gain profound insights into local preferences, enabling the curation of assortments tailored to each store’s unique customer base. This data-driven approach enhances customer satisfaction by aligning offerings with local tastes and minimizing excess inventory, optimizing resource allocation and reducing carrying costs.
Benefits of AI in store replenishment
The integration of AI in store replenishment processes brings forth numerous benefits that significantly enhance operational efficiency, customer satisfaction, and overall business performance:
- Accurate demand forecasting: AI-driven demand forecasting improves accuracy by analyzing historical data, trends, and external factors, reducing stockouts and excess inventory, resulting in better customer satisfaction and cost savings.
- Optimal inventory levels: AI optimizes inventory levels by considering multiple variables, preventing understocking and overstocking situations, minimizing holding costs, and maximizing capital utilization.
- Real-time decision-making: AI processes real-time data, enabling quicker and more informed decisions, leading to timely replenishment and responsiveness to changing demand patterns.
- Efficient supplier collaboration: AI facilitates efficient communication and collaboration with suppliers, resulting in better alignment between supply and demand, reduced lead times, and improved order accuracy.
- Automation and time savings: AI automates manual tasks like reorder point calculation, purchase order generation, and data analysis, freeing up employees to focus on higher-value tasks.
- Data-driven insights: AI extracts insights from diverse data sources, aiding retailers in making informed decisions, identifying trends, and adapting to market changes.
- Reduced wastage: Accurate demand forecasting and optimized inventory levels minimize the risk of wastage due to overstocking or expired products, reducing costs and environmental impact.
- Enhanced customer satisfaction: By ensuring products are consistently available and accurately priced, AI-driven replenishment improves customer experience and loyalty.
- Adaptive learning: AI algorithms continually learn from new data, adapting to changing market dynamics and improving accuracy over time, leading to more effective replenishment strategies.
- Streamlined operations: AI reduces manual errors, streamlines processes, and enables better resource allocation, resulting in smoother and more efficient operations.
- Scalability: AI-powered systems can easily scale to handle larger product catalogs, multiple store locations, and increased transaction volumes.
- Optimized shelf placement: AI analyzes store layouts and customer behavior to suggest optimal shelf placements, enhancing product visibility and increasing sales.
- Strategic decision-making: AI provides retailers with data-driven insights that enable them to make strategic decisions about product assortment, pricing, and inventory management.
- Competitive advantage: Retailers leveraging AI for store replenishment gain a competitive edge by staying ahead of market trends, responding rapidly to changes, and optimizing resource utilization.
How does LeewayHertz’s generative AI platform streamline store replenishment workflows?
LeewayHertz’s generative AI platform, ZBrain, plays a transformative role in streamlining store replenishment processes, optimizing inventory levels, and enhancing overall store efficiency. As a comprehensive, enterprise-ready platform, ZBrain empowers businesses to design and implement applications tailored to their specific operational requirements. The platform uses clients’ data, whether in the form of text, images, or documents, to train advanced LLMs like GPT-4, Vicuna, Llama 2, or GPT-NeoX for developing contextually aware applications capable of performing diverse tasks.
In the realm of in-store replenishment, businesses encounter obstacles, including constrained inventory visibility, challenges in demand forecasting, inaccuracies in stock levels, suboptimal restocking schedules, and the need to adapt to evolving customer preferences swiftly. ZBrain effectively addresses these challenges through its distinctive feature called “Flow,” which provides an intuitive interface that allows users to create intricate business logic for their apps without the need for coding. Flow’s easy-to-use drag-and-drop interface enables the seamless integration of large language models, prompt templates, and other genAI models into your app’s logic for its easy conceptualization, creation, or modification.
To comprehensively understand how ZBrain Flow works, explore this resource that outlines a range of industry-specific Flow processes. This compilation highlights ZBrain’s adaptability and resilience, showcasing how the platform effectively meets the diverse needs of various industries, ensuring enterprises stay ahead in today’s rapidly evolving business landscape.
ZBrain’s robust applications optimize the store replenishment process by transforming complex data into actionable insights. This ensures maintaining optimal inventory levels, reducing costs, easing manual workloads, boosting productivity, and improving operational efficiency. Dive into the next segment, elucidating how ZBrain enhances efficiency in in-store replenishment processes within the retail sector through tailored recommendations, optimizing the entire replenishment workflow.
AI-driven in-store replenishment strategies in retail
ZBrain transforms in-store replenishment processes in the retail sector through an automated approach. It initiates the process by autonomously aggregating essential data from diverse sources, encompassing stock levels, promotion details, customer purchase patterns, and product demand data. Through a thorough Exploratory Data Analysis (EDA), it identifies and corrects anomalies, unveiling insights into stock movement patterns, sales trends, and potential replenishment needs. Historical sales, inventory, and supply chain data undergo embedding, forming the foundation for ZBrain’s ability to deliver precise recommendations for optimized inventory management. When a replenishment decision is necessary, ZBrain accesses pertinent data, incorporating user-specific queries and processing information through the chosen Language Model (LLM). The LLM carefully evaluates query requirements and available data to generate actionable replenishment strategies. The generated recommendations then undergo detailed parsing, ensuring the delivery of precise directives regarding which products to restock, quantities, and optimal timings. ZBrain’s approach ensures retailers receive timely replenishment strategies aligned with store needs, facilitating well-stocked shelves while minimizing excess inventory.
ZBrain applications enable retail organizations to enhance in-store replenishment processes through real-time, data-driven insights, improved demand forecasting accuracy, optimized shelf space utilization, and customized replenishment strategies aligned with customer preferences. This results in minimized stockouts, improved supply chain visibility, and substantial cost savings, ultimately driving increased efficiency and operational enhancements. Delve into this detailed flow to grasp how ZBrain improves in-store replenishment processes within the retail sector, presenting a more precise and simplified approach to optimizing inventory management and maximizing sales efficiency.
AI technologies used in store replenishment
AI technologies play a pivotal role in modernizing store replenishment processes. Some key AI technologies used in store replenishment include:
Machine Learning (ML): ML algorithms can analyze historical sales data, demand patterns, and external factors to predict future demand accurately. They can also optimize inventory levels by considering various variables and adjusting replenishment strategies over time based on real-time feedback.
Predictive analytics: Predictive analytics leverages historical and real-time data to forecast demand, stockouts, and optimal inventory levels. This aids retailers in making informed decisions about when and how much to reorder.
Natural Language Processing (NLP): NLP enables systems to understand and process human language, facilitating communication between retailers and suppliers. It can automate the generation of purchase orders, allowing for faster and more accurate order placement.
Data integration platforms: AI-powered platforms can integrate data from multiple sources, such as sales records, inventory databases, and market data, to provide a comprehensive view of replenishment needs and trends.
Reinforcement learning: This type of machine learning can optimize replenishment strategies through trial-and-error learning, adapting to changing market conditions and improving decisions over time.
Collaborative filtering: Collaborative filtering techniques use customer behavior data to suggest complementary products, aiding retailers in cross-selling and effectively managing inventory levels for related items.
Robotics and automation: AI-powered robots can be used for efficient inventory counting, sorting, and restocking, reducing the need for manual intervention and streamlining the replenishment process.
Computer vision: Computer vision technologies can analyze shelf conditions and product availability, enabling retailers to replenish shelves and prevent stockouts proactively.
Time series analysis: AI can perform time series analysis on historical data to identify recurring patterns and seasonal trends, helping retailers prepare for fluctuations in demand.
Launch your project with LeewayHertz!
Leverage AI tools for store replenishment and enhance your retail or e-commerce business. Reach out to us now to explore tailor-made solutions that align seamlessly with the unique needs of your business.
How to implement AI in store replenishment?
Implementing AI in store replenishment is a strategic endeavor that requires careful planning, execution, and ongoing refinement. Here’s a comprehensive guide on how to effectively implement AI in store replenishment:
Step 1: Evaluate current processes and needs
- Conduct a comprehensive assessment of existing store replenishment procedures.
- Identify pain points, inefficiencies, and areas requiring improvement.
- Define specific business needs and objectives for integrating AI into store replenishment.
Step 2: Establish objectives and success metrics
- Clearly outline objectives for implementing AI in store replenishment.
- Define measurable success metrics, such as inventory optimization, stockout reduction, forecast accuracy improvement, and operational efficiency enhancement.
- Use these metrics to track progress and evaluate the effectiveness of the AI system.
Step 3: Integrate and prepare data
- Integrate AI-powered replenishment systems with current inventory management software and point-of-sale systems.
- Ensure seamless data flow and compatibility to enable real-time access to essential information.
- Cleanse and preprocess data to enhance its quality and suitability for AI algorithms.
Step 4: Choose AI algorithms and models
- Select AI algorithms and models that align with store replenishment requirements.
- Consider factors like demand forecasting methods, inventory optimization techniques, and decision-making processes for replenishment.
- Tailor AI solutions to suit specific business needs and objectives.
Step 5: Train and optimize
- Train AI algorithms using historical data to develop accurate predictive models.
- Fine-tune algorithms based on feedback loops and performance evaluations.
- Adjust parameters and algorithms to accommodate changing market dynamics and seasonal variations.
Step 6: Implement and integrate
- Deploy AI-powered replenishment systems into operational workflows.
- Ensure seamless integration with existing processes and systems.
- Provide training and support to employees to familiarize them with the AI system and its functionalities.
Step 7: Monitor and evaluate continuously
- Regularly monitor the performance of AI replenishment systems using predefined success metrics.
- Analyze results, identify patterns, and assess the impact on key performance indicators (KPIs) such as inventory turnover and customer satisfaction.
- Make necessary adjustments and refinements to optimize system performance and achieve desired outcomes.
Step 8: Foster iterative improvement and innovation
- Promote a culture of continuous improvement and innovation.
- Encourage experimentation with new AI technologies, methodologies, and best practices.
- Gather feedback from stakeholders, customers, and employees to identify opportunities for enhancement and innovation in store replenishment processes.
By following these steps, retailers can successfully implement AI in store replenishment, driving operational efficiency, optimizing inventory management, and delivering superior customer experiences.
Exploring the future of AI in store replenishment
In the constantly changing world of retail, the future of store replenishment is undergoing a significant transformation, largely driven by the continuous progress of AI technologies. Let’s take a look at what lies ahead, where innovation meets the needs of consumers, fundamentally altering how we manage our inventory.
- Advanced predictive analytics: AI will utilize sophisticated predictive analytics tools, deep learning algorithms, and predictive modeling techniques to forecast demand more accurately and in greater detail than ever before. This means that retailers can anticipate consumer needs more precisely, reducing overstocking or stockouts and optimizing inventory levels.
- Autonomous replenishment systems: AI-powered systems, integrated with IoT devices, will enable stores to automatically reorder inventory based on real-time demand signals and inventory levels. These systems can also adjust pricing dynamically and optimize product placements within the store layout. Essentially, this allows for a more efficient and responsive supply chain management process.
- Collaborative ecosystems: Collaboration between retailers, suppliers, and technology providers will become more common. Through integrated ecosystems, stakeholders will share data, insights, and resources to improve supply chain efficiency, enhance inventory visibility, and create value for all parties involved. This collaborative approach can lead to more streamlined operations and better decision-making across the supply chain.
- Augmented Reality(AR) and virtual shopping: AI-driven AR and virtual shopping experiences will redefine how consumers interact with products, both online and in-store. These technologies enable personalized virtual try-ons, tailored product recommendations, and immersive shopping experiences. By integrating AI into these experiences, retailers can better understand consumer preferences and offer more personalized and engaging shopping experiences.
Endnote
The integration of AI in store replenishment stands as a transformative advancement in the retail landscape. The convergence of sophisticated ML algorithms, real-time data analytics, and automation has not only streamlined the replenishment process but has also ushered in a new era of efficiency and customer satisfaction. With AI-driven predictive models accurately forecasting demand patterns and optimizing inventory levels, retailers can significantly reduce stockouts, minimize excess inventory, and ultimately enhance profitability. As AI continues to evolve, we can expect even more innovative solutions that will reshape how retailers manage and optimize their inventory, further cementing AI’s role as a driving force in the future of retail.
Boost your retail/e-commerce business with LeewayHertz’s AI-driven solutions. Contact us now to discover how we can tailor our AI expertise to your unique business needs.
Start a conversation by filling the form
All information will be kept confidential.
Insights
Generative AI: A Comprehensive Tech Stack Breakdown
A well-implemented generative AI tech stack can help businesses streamline their workflows, reduce costs, and improve overall efficiency.
How to create a generative video model?
Generative video models are machine learning algorithms generating new video data based on patterns learned from training datasets. Explore more about it!
How to build a generative AI model for image synthesis?
With tools like Midjourney and DALL-E, image synthesis has become simpler and more efficient than before. Dive in deep to know more about the image synthesis process with generative AI.