Machine learning for customer segmentation: Unlocking new dimensions in marketing
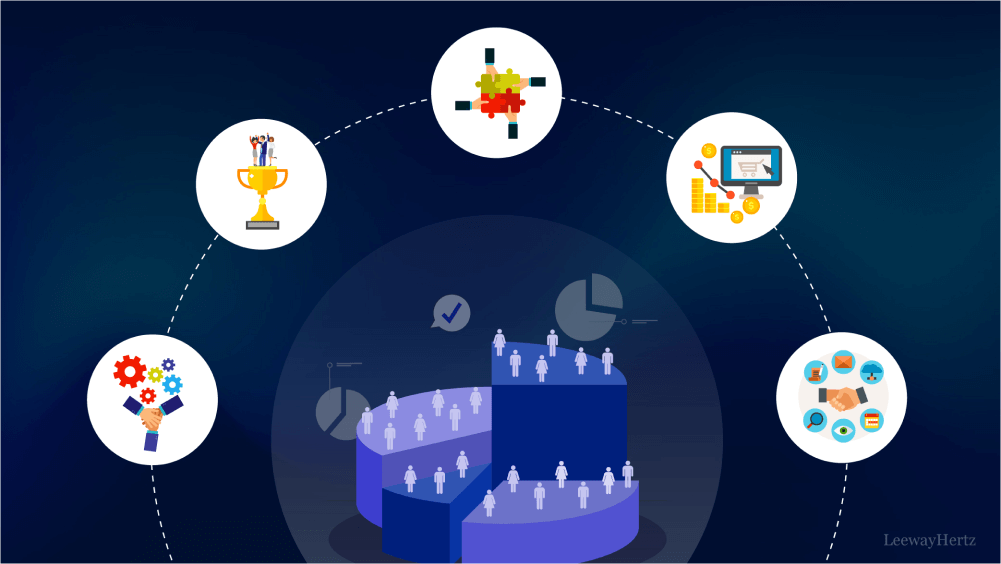
Understanding your customers is paramount for business success in today’s data-driven world. Whether you are an IT giant, a tech startup, or a local retail provider, customer segmentation can be a game-changer. It allows you to tailor your marketing strategies, personalize user experiences, and optimize resource allocation.
But what exactly is customer segmentation? Imagine you are at a bustling farmer’s market exploring a variety of fruits. Each fruit has unique characteristics: sweetness, color, texture, and taste. Similarly, your customer base comprises diverse individuals with distinct preferences, behaviors, and needs. Customer segmentation is the art of grouping these individuals based on shared attributes. It’s like sorting those fruits into baskets: apples together, oranges in another, and maybe a special basket for exotic dragon fruit. By doing so, you gain insights into your audience, enabling targeted marketing efforts.
Was this example explanatory? Let’s delve into the landscape of customer segmentation, a strategic methodology poised to transform how businesses connect with their audience. This powerful approach divides a diverse customer base into distinct groups based on common characteristics. While traditional segmentation methods have proven useful, the integration of machine learning methods has transformed the precision and efficiency of this process.
Email marketing has remained one of the core functions for marketers. The following stats underscore the importance of segmentation across email marketing campaigns.
- Segmented campaigns can lead to a 760% increase in revenue (DMA).
- 39% of email marketers that practice list segmentation observe improved open rates, and 24% see increased sales leads (eMarketer).
- 56% of individuals choose to unsubscribe from emails when the content ceases to be relevant to their interests. (Chadwick Martin Bailey).
This article provides a comprehensive guide to building a machine-learning model for customer segmentation. We delve into the critical steps in building an ML model for customer segmentation, its importance in sales and marketing, and how to evaluate its performance.
- What is customer segmentation?
- Exploring the types of customer segmentation
- Why is customer segmentation important?
- The role of machine learning for customer segmentation and its edge over traditional segmentation methods
- How to build a machine learning model for customer segmentation?
- Evaluating the effectiveness of customer segmentation models
- Leverage LeewayHertz’s expertise for customer segmentation model development
What is customer segmentation?
At its core, customer segmentation is the process of dividing a broad audience into smaller, more manageable segments. These segments, often called cohorts or groups, share common traits that enable businesses to tailor their strategies, communication, and offerings effectively. The overarching goal is to treat each segment as unique, understanding and addressing their specific requirements.
The primary objective of this practice is to interact with customers within each segment strategically, maximizing their value to the business. The concept revolves around recognizing that not all customers are the same and tailoring interactions to meet each customer group’s unique needs and preferences. For example – Imagine a fashion retailer looking to re-engage customers who haven’t shopped in the last 45 days. By analyzing past purchases, the retailer discovers a preference for seasonal clothing during sales and an inclination towards accessory bundles with discounts. Armed with this insight, the marketing team launched a targeted campaign offering personalized discounts on seasonal wear and exclusive deals on accessories. Campaign result? A successful reactivation drove renewed interest, increased purchases, and enhanced customer engagement.
What is customer segmentation analysis?
Customer segmentation analysis is the intricate process of unearthing insights defining specific customer segments. In this analysis, marketers and brands delve into data to determine the most effective campaigns, offers, or products for communication with each segment. This strategic approach is vital for crafting personalized and targeted initiatives, ensuring that marketing efforts are finely tuned to resonate with each customer group’s distinct characteristics and behaviors.
Exploring the types of customer segmentation
Customer segmentation is a pivotal strategy that empowers businesses to understand and cater to the diverse needs of their audience. In this section, we will delve into the different types of customer segmentation, each offering a unique lens through which businesses can gain insights into their audience.
Demographic segmentation
Demographic segmentation involves categorizing customers based on attributes such as age, gender, income, education, and occupation. This segmentation method provides a fundamental understanding of the customers and allows businesses to tailor their strategies to specific demographic profiles. For instance, a clothing brand may target a younger demographic with trendier designs, while a financial institution might tailor its services differently for various income brackets.
- Age groups: Are your customers mostly millennials, Gen Z, or baby boomers? Each group has distinct preferences and behaviors. For instance, millennials might crave convenience, while baby boomers value tradition.
- Gender: Understanding the gender distribution helps tailor your messaging. A skincare brand might emphasize different benefits for men and women.
- Location: Geographic segmentation matters. Urban dwellers might seek speed and efficiency, while rural customers appreciate personalized service.
- Income levels: High-income customers may prioritize luxury, while budget-conscious shoppers seek value.
Geographic segmentation
Geographic segmentation divides customers based on their physical location or region. This segmentation considers factors such as country, city, climate, or even local cultural preferences. Businesses can then tailor their products, services, and marketing campaigns to align with the unique characteristics of each geographic segment.
- Urban vs. rural: Urban customers may prioritize convenience, while rural customers value community.
- Climate considerations: Tropical regions have different needs than cold climates.
- Cultural nuances: Respect cultural differences. What works in Tokyo might not resonate in New York.
Psychographic segmentation
Psychographic segmentation delves into customers’ lifestyles, values, interests, attitudes, and personality traits. This type of segmentation goes beyond the ‘what’ and ‘where,’ focusing on the ‘why’ behind customer choices. Understanding customers’ psychographics allows businesses to create targeted marketing campaigns that resonate with specific groups on a deeper level. For example, a travel brand might cater to adventure-seeking individuals with a penchant for unique experiences, crafting personalized travel packages that align with their values and preferences.
- Interests and hobbies: What do your customers love? Are they adventure seekers or bookworms?
- Attitudes: Understand their beliefs and attitudes. Are they eco-conscious, tech-savvy, or family-oriented?
- Personality traits: Are they risk-takers, introverts, or extroverts? Tailor your messaging accordingly.
Behavioral segmentation
Behavioral segmentation analyzes customers based on their behaviors, such as purchasing patterns, brand loyalty, product usage, or responses to marketing stimuli. Businesses can tailor their strategies to match customer preferences and habits by understanding how customers interact with products and services. An e-commerce platform may categorize customers based on their frequency of purchase, average order value, or response to promotional offers, enabling personalized recommendations and targeted marketing efforts.
- Purchase behavior: Who are your frequent buyers? Who makes occasional purchases? Analyze transaction history to identify patterns.
- Engagement: Do some customers interact more with your brand? Are they active on social media, sign up for newsletters, or participate in loyalty programs?
- Usage frequency: Segment based on usage frequency for subscription services or products. Are some customers power users, while others are occasional users?
The diverse landscape of customer segmentation provides businesses with a multifaceted approach to understanding and engaging with their audience. By leveraging demographic, geographic, psychographic, and behavioral segmentation, businesses can navigate the intricacies of their customer base, ultimately delivering more personalized and effective strategies that resonate with specific customer segments. This nuanced understanding not only enhances customer satisfaction but also positions businesses for sustained success in today’s competitive market.
The following table summarizes the detailed types of different segmentation approaches used:
Refine Customer Segmentation with AI Expertise!
Explore LeewayHertz’s AI consulting services to fine-tune your customer segmentation strategies and boost business outcomes.
Segmentation type/criteria | Example | Impact on marketing strategy |
Geographic segmentation | Targeting users based on location, e.g., offering winter coats in August to Australians. | Tailor campaigns to specific regions, optimizing product relevance for local audiences. |
Gender | Tailoring products and experiences based on gender-specific shopping behaviors. | Develop targeted campaigns catering to distinct gender preferences and interests. |
Age | Understanding how different age groups interact with products, targeting campaigns accordingly. | Adjust marketing messages and products to resonate with the preferences of specific age groups. |
Profession segmentation | Targeting audiences based on their professions or jobs, offering personalized content. | Craft tailored content and promotions catering to the professional segment’s unique needs and interests. |
Device types | Segmenting audiences based on the type of devices they use, optimizing offers for mobile users. | Enhance user experience by tailoring campaigns to specific devices, maximizing conversion rates. |
Cart abandonment | Retargeting users who abandoned their carts with popups, email flows, and text messages. | Recover potential sales by targeting users with personalized incentives based on abandoned cart behavior. |
Product type or collection | Retargeting based on past purchases, offering similar or complementing products. | Increase average order value by suggesting products that align with customers’ previous purchase history. |
Lifestyle | Using behavioral and demographic factors to identify target customers with specific lifestyles. | Create campaigns that align with customers’ lifestyles, establishing a deeper connection with the audience. |
Interest segmentation | Targeting audiences with related interests around a product, common in athletic brands. | Enhance engagement by aligning marketing messages with customers’ specific interests and hobbies. |
Family situation | Segmenting audiences based on marital status, family size, and children’s age groups. | Tailor marketing messages to address customer’s unique needs and preferences in specific family stages. |
Top purchasers | Segmenting based on loyalty, targeting customers who have purchased multiple times. | Foster loyalty by providing exclusive rewards and incentives to top purchasers, maximizing customer retention. |
Referred customers | Segmenting based on whether customers were referred, focusing on improving referral sales. | Encourage referrals by creating targeted campaigns for customers who have been referred to your brand. |
Re-engage inactive customers | Using psychographic segmentation, segment and re-engage customers who haven’t made purchases in a while. | Revitalize customer relationships by addressing inactive segments with personalized re-engagement strategies. |
Best reviewers | Segmenting based on customers who left reviews, acknowledging their contribution and encouraging engagement. | Recognize and appreciate customers who contribute reviews, fostering a positive relationship with brand advocates. |
Welcome flow for new customers | Initiating a welcome flow for new visitors, gradually increasing customer engagement. | Create a positive first impression by welcoming new customers with exclusive offers and targeted communication. |
Frequent users | Targeting loyal customers and brand ambassadors, encouraging organic growth, and increasing online reach. | Cultivate organic growth by engaging with and rewarding loyal customers and expanding brand reach online. |
Why is customer segmentation important?
Understanding your customers isn’t a one-size-fits-all endeavor. Customer segmentation brings a strategic approach that slices and dices your audience into meaningful subgroups. Let’s explore why this practice is crucial for your business success:
Enhanced customer relationship and brand loyalty
Customer segmentation involves grouping existing and potential customers based on shared characteristics. When you segment customers into different classes, you gain insights into their needs, preferences, and buying patterns. Here’s why it matters:
- Tailored marketing messages: Segmentation allows you to align your marketing messages precisely with what each customer seeks.
- Effective communication channels: Segmentation reveals which channels resonate with specific customer groups. Whether it’s email, social media, or personalized offers, you can engage effectively.
Enhanced customer experience and sales
Did you know that cart abandonment is a trillion-dollar problem? Almost 70% of desktop users leave their carts before purchasing, which is even higher for mobile users at 86%. Customer segmentation isn’t just about data—it’s about delivering value. Here’s how it impacts your bottom line:
- Customized offerings: With segmentation, you’ll know what customers need, when they need it, and why. Fine-tuning your marketing messages ensures users receive ads or promotions precisely aligned with their requirements. Happy customers make more purchases.
- Resource efficiency: Time is money. By focusing on the right segments, your business saves resources. Instead of spraying generic messages, you invest where it counts. Whether seasonal adjustments or personalized recommendations, efficiency leads to increased sales.
Strategic decision-making
Customer segmentation isn’t just for marketers—it transforms your entire business strategy:
- Investment prioritization: Like a wise gardener tending to specific plants, segmentation helps you decide where to invest. High-value segments deserve extra attention. Allocate resources strategically based on potential returns.
- Product development: Segmentation guides product development. Whether it’s launching a loyalty program, introducing a new feature, or tweaking existing offerings, you’ll know whom to target.
Enhanced customer understanding
Customer segmentation acts as a magnifying glass, revealing intricate details about your audience. Here’s how:
- Data-driven insights: By analyzing demographics, purchase history, online behavior, and preferences, you gain a 360-degree view of different customer segments. For instance, a retail brand might discover a segment of price-conscious shoppers who prefer online discounts. With this knowledge, the business can tailor campaigns and product offerings specifically for this savvy group. Segmentation is an instrumental tool to avoid possible customer churn with proactive actions.
- Behavioral patterns: Segmentation unveils behavioral nuances. Do some customers abandon carts frequently? Are others loyal repeat buyers? Understanding these patterns helps you address pain points and create targeted solutions.
Improved customer engagement
72% of customers switch companies after a single negative experience. More surprisingly, 52% of dissatisfied customers won’t keep their dissatisfaction to themselves. They will ensure their friends and circle hear about it, actively discouraging them from purchasing the same brand. That’s why appropriate customer engagement is the heartbeat of any business. Segmenting customers enables businesses to communicate with them more meaningfully and relevantly. Understanding each segment’s specific needs, pain points, and aspirations allows for crafting messages that resonate on a personal level.
- Higher interaction levels: Customers are more likely to engage when they receive relevant content. Personalized communication boosts interaction, whether it’s opening emails, clicking on ads, or participating in surveys.
Targeted marketing strategies
Efficient allocation of marketing resources is a primary advantage offered by customer segmentation. Businesses can prioritize and tailor marketing efforts by identifying segments with the highest potential value. An e-commerce company, for instance, might discover a segment of frequent buyers who spend significantly more than the average customer. Dedicating targeted marketing campaigns, loyalty programs, and personalized recommendations to this segment amplifies revenue and maximizes return on investment.
- Segment-specific tactics: Each segment requires a unique approach. Whether it’s social media ads, influencer collaborations, or referral programs, tailor your strategies to resonate with specific groups.
- Resource efficiency: Customer segmentation helps focus on high-value segments. For example, an e-commerce company might discover a segment of big spenders. Targeted marketing campaigns, loyalty programs, and personalized recommendations yield better results.
Uncovering new market opportunities
Customer segmentation is not merely a tool for understanding existing customers but also a compass for navigating uncharted market territories. By scrutinizing data and identifying patterns, businesses may uncover segments overlooked or underserved by competitors. These undiscovered segments often harbor unique needs or preferences that present golden opportunities for innovation and market differentiation.
- Niche segments: Segmentation reveals hidden gems. Perhaps there’s an underserved market waiting for your product. Dive into niche segments—early adopters, eco-conscious consumers, or tech enthusiasts.
- Innovation pathways: Understanding segments sparks creativity. It helps leverage these insights to innovate—new product lines, partnerships, or services.
The role of machine learning for customer segmentation and its edge over traditional segmentation methods
In the customer segmentation approach, machine learning integration has emerged as a game-changer, elevating the precision and efficiency of this strategic practice. Machine learning excels in predictive analytics, leveraging advanced algorithms to forecast future customer behaviors with unparalleled accuracy. Unlike traditional approaches that rely heavily on historical data, machine learning models can analyze vast datasets and identify subtle patterns, enabling businesses to predict customer preferences, purchasing trends, and potential churn. This predictive precision empowers businesses to tailor strategies, optimizing customer experiences and resource allocation proactively.
ML models, characterized by their dynamic adaptability, continuously learn from new data inputs, allowing real-time adjustments to segmentation strategies. Traditional approaches, typically static and based on fixed criteria, face challenges adapting to evolving customer behaviors. Machine learning’s iterative learning process ensures businesses stay ahead of shifting preferences and emerging trends, fostering a proactive approach to customer engagement. This adaptability positions ML models as a powerful tool for businesses navigating the landscape of consumer dynamics, offering a level of agility that traditional methods struggle to match.
According to the latest research, 52% of customers demand fast, personalized, and interactive support. Machine learning enables businesses to achieve unparalleled levels of personalization by analyzing vast datasets to discern intricate patterns in customer behavior. This capability helps deliver highly personalized recommendations and experiences, even at scale. Traditional approaches, often manual and resource-intensive, need to be revised to handle the complexity of personalized segmentation.
The automation capabilities of machine learning streamline the segmentation process, enhancing operational efficiency for businesses. While traditional approaches involve manual sorting and categorization, supervised and unsupervised ML algorithms can automatically analyze complex patterns and categorize customers based on multifaceted criteria. This automation not only accelerates the segmentation process but also allows businesses to handle large and diverse datasets with precision and speed. The result is a more efficient allocation of resources, optimized targeting, and campaigns that resonate with specific customer segments, providing matchless operational efficiency.
The role of machine learning for customer segmentation is transformative, offering precision, adaptability, personalization at scale, operational efficiency, and enhanced risk mitigation. Compared to traditional approaches, machine learning stands out as a dynamic and sophisticated tool, providing businesses with the agility and insights needed to navigate the complexities of the modern market landscape.
Refine Customer Segmentation with AI Expertise!
Explore LeewayHertz’s AI consulting services to fine-tune your customer segmentation strategies and boost business outcomes.
How to build a machine learning model for customer segmentation?
Customer segmentation is a powerful technique that helps businesses understand their customer base better and tailor their marketing strategies accordingly. Machine learning for customer segmentation involves the application of advanced algorithms to analyze and categorize customers based on various features and behaviors. Here’s a step-by-step guide on how to build an ML model for customer segmentation:
Design a proper business case as your first step
Before diving into the technical aspects of creating a customer segmentation model, it’s essential to establish a clear business context. Here’s how to approach this step:
Define your objectives
- Understand why you need customer segmentation. What specific business goals are you trying to achieve?
- Examples of segmentation objectives:
- Improve targeted marketing efforts.
- Enhance customer experience.
- Optimize resource allocation.
- Identify high-value segments.
Identify key stakeholders
- Determine who will benefit from the segmentation results.
- Involve relevant teams such as marketing, sales, product development, and customer support.
Gather relevant information
- Collect data on your existing customer base.
- Understand the context in which segmentation will be applied (e.g., retail, e-commerce, finance).
- What specific insights do you seek from customer segmentation?
- How will the segments impact decision-making?
- Are there any constraints (e.g., budget, data availability)?
Align with business strategy
- Ensure that the segmentation aligns with your overall business strategy.
- Consider long-term implications and scalability.
Remember, a well-defined business case sets the foundation for successful customer segmentation. It ensures that your efforts are purposeful and aligned with organizational goals.
Data collection and preprocessing
Once your problem statement is ready, the next step is to gather relevant data and transform it into the desired format.
Collect relevant data
Gather data about your customers, including features like age, spending, transaction history, and any other relevant attributes. Sources may include:
- Transactional records (purchases, returns).
- Customer profiles (demographics, contact information).
- Interaction data (website visits, app usage).
- Surveys or feedback forms.
- Social media interactions.
Data quality
- Ensure the data is accurate and comprehensive.
- Ensure data accuracy and completeness.
- Address missing values or outliers.
Data cleaning
- Remove inconsistencies, errors, and duplicates.
- Handle missing values (impute or remove).
- Standardize formats (e.g., unify date formats).
Data integration
- Combine data from different sources into a single dataset.
- Resolve schema differences (e.g., merge customer profiles and purchase history).
Data transformation
- Convert categorical variables (e.g., gender, product categories) into numerical representations.
- Normalize numerical features (e.g., scale spending amounts).
Data reduction
- Reduce dimensionality if needed (e.g., using Principal Component Analysis).
- Select relevant features for segmentation.
Feature selection
Selecting the right features is crucial for effective customer segmentation. By focusing on meaningful attributes such as age, spending behavior, purchase frequency, and geographic location, you enhance the accuracy and relevance of your segmentation model. This step ensures that the chosen features align with your business objectives, providing valuable insights into customer behavior and preferences.
- Select the most meaningful features for segmentation.
- Consider features like age, spending behavior, purchase frequency, and geographic location.
Algorithm selection
Choosing the appropriate algorithm is pivotal in the customer segmentation process. Each algorithm has its strengths and is suited for specific scenarios. In most cases, customer segmentation is considered as an unsupervised learning problem.
Common algorithms for customer segmentation include:
- K-means clustering: Ideal for grouping similar customers into clusters based on feature similarity.
- Hierarchical clustering: Creates a tree-like structure of clusters, offering insights into hierarchical relationships.
- DBSCAN (Density-based spatial clustering of applications with noise): Identifies dense regions in the data, useful for detecting clusters with varying shapes and densities.
Model training
Utilizing Python libraries like Scikit-Learn or Keras, model training is a critical step in customer segmentation. By fitting the chosen algorithm to preprocessed data and experimenting with hyperparameters, you enhance the model’s ability to identify meaningful clusters within your customer base. Effective training enhances the model’s accuracy, leading to more reliable and actionable segmentation results. Experimenting with hyperparameters allows customization, adapting the model to diverse business scenarios.
- Use Python libraries like Scikit-Learn or Keras.
- Choose the appropriate algorithm and fit it to your preprocessed data.
- Experiment with different hyperparameters (e.g., number of clusters for K-means).
Hyperparameter tuning
After training the model, the next crucial step is hyperparameter tuning. This involves finding the most effective set of hyperparameters for the chosen algorithm to achieve accurate and meaningful customer segmentation.
K-means optimization: Determining the optimal value for k is essential when using K-means clustering. The inertia, a measure of how spread out the clusters are, is a key optimization parameter.
Elbow method: Employing the elbow method aids in selecting the optimal k value. By observing the inertia and identifying the point where its decrease stabilizes (elbow point), businesses can make an informed decision on the number of clusters.
Remember that hyperparameter tuning is dynamic, and adjustments may be needed as your customer data evolves or business objectives change. Regular refinement ensures that your segmentation model remains effective and aligned with your goals.
Interpretation
Analyzing the resulting clusters is fundamental to deriving actionable insights. Understanding each segment’s characteristics, patterns, and differences empowers businesses to make informed decisions. Assigning meaningful labels further refines the interpretation process.
Understand the clusters
- Analyze the resulting clusters.
- Explore the characteristics of each segment.
- Identify patterns and differences.
Assign meaningful labels
- Label each cluster with descriptive names (e.g., “High-Value Customers,” “Budget Shoppers,” “Inactive Users”).
Clarity on segment characteristics aids in developing targeted strategies, ensuring marketing efforts resonate with specific customer groups. Assigning descriptive labels facilitates strategic alignment, enabling seamless integration of segmentation insights into broader business strategies.
Visualization and insights
Utilizing visualization tools like Seaborn, Matplotlib, or Plotly Express enhances the interpretability of segmentation results. Scatter plots, bar charts, and heat maps visually represent customer distribution across segments.
Extract insights
- Explore differences between segments.
- Identify opportunities for targeted marketing campaigns.
- Personalize communication based on segment characteristics.
Remember that customer segmentation is an ongoing process. Regularly update and refine your model as your business evolves.
Evaluating the effectiveness of customer segmentation models
Once a customer segmentation model is built using machine learning methods, evaluating their effectiveness becomes paramount. This assessment helps businesses ascertain if the segmentation approach achieves its intended goals and furnishes meaningful insights for decision-making. In this section, we delve into key factors and metrics for evaluating the effectiveness of customer segmentation models.
Cohesion and separation
- Cohesion: Evaluate the internal similarity within each segment.
- Separation: Assess the dissimilarity between different segments.
- Ideal segments are internally cohesive and well-separated, ensuring distinct characteristics for effective targeting. For instance, a segment identifying frequent buyers should exhibit similar purchasing patterns, setting it apart from other segments.
Predictive power
- Assess the model’s ability to predict customer behavior accurately.
- The model’s efficacy is measured through precision, recall, and F1 score for churn prediction by comparing predicted outcomes with actual results.
- A robust segmentation model provides reliable foresight into customer actions.
Actionability
- Effective segmentation should offer actionable insights for tailored strategies.
- Segments must guide specific actions to engage customers differently.
- For instance, identifying price-sensitive customers allows businesses to offer targeted discounts or promotions.
Stability over time
- Evaluate the model’s stability amid changing market dynamics and customer behaviors.
- Consistency in segment assignments and characteristics over time signifies stability.
- Any significant changes may necessitate recalibration or reassessment of the segmentation model.
Business impact
- Analyze the impact of segmentation models on key performance indicators (KPIs).
- Measure outcomes like increased sales, enhanced customer satisfaction, or improved retention rates.
- For personalized marketing campaigns, compare KPIs before and after implementation to gauge effectiveness.
In conclusion, thoroughly evaluating models is essential for effectively leveraging machine learning for customer segmentation. Businesses can make informed, data-driven decisions by scrutinizing factors such as cohesion, separation, predictive power, actionability, stability over time, and business impact. This optimization ensures that marketing strategies are tailored and customer experiences are enhanced for sustainable business growth.
Leverage LeewayHertz’s expertise for customer segmentation model development
At LeewayHertz, we offer a comprehensive and tailored approach to building machine learning models specifically designed for customer segmentation. Our expertise lies in delivering innovative solutions that align with your business goals and leverage advanced ML techniques to optimize customer understanding.
We understand your organization’s unique needs, challenges, and objectives. We then gather insights into your business processes, customer base, and goals for effective customer segmentation through detailed consultations.
Leveraging our deep understanding, we develop a customized ML strategy focused on customer segmentation. This strategy encompasses key considerations such as data requirements, algorithm selection, and integration with your existing systems. We specialize in crafting ML models tailored to your business’s specific needs. By incorporating your proprietary data, we fine-tune the models to accurately segment customers based on behavior, preferences, and demographics.
ML models are dynamic, and customer behaviors evolve. We implement mechanisms for continuous refinement, regularly updating the models to adapt to changing customer trends, ensuring the longevity and relevance of the segmentation strategies. Our commitment extends beyond the implementation phase. We provide continuous support for your ML initiatives by addressing any issues, continuously monitoring model performance, and incorporating feedback to enhance the effectiveness of the segmentation model.
Endnote
In summary, customer segmentation, coupled with the transformative power of machine learning, stands as a cornerstone for modern businesses seeking success in a dynamic market. As businesses navigate the evolving landscape of customer dynamics, the importance of customer segmentation becomes more apparent than ever. The strategic advantages, including enhanced customer relationships, personalized marketing strategies, and resource optimization, position segmentation as a core aspect of success. Machine learning’s transformative role adds a layer of sophistication, enabling businesses to navigate the complexities of the market with unparalleled precision and agility.
This guide navigates through the nuances of segmentation, emphasizing its pivotal role in enhancing customer relationships, streamlining marketing strategies, and optimizing resources. With machine learning at its forefront, businesses gain a competitive edge by harnessing predictive analytics and operational efficiency, underscoring the significance of a customer-centric approach in today’s data-driven landscape.
Unlock the potential of AI and ML to elevate your marketing strategy through data-driven insights, enabling smarter decisions and strategic customer segmentation. Contact LeewayHertz experts for all your AI consulting and development needs.
Start a conversation by filling the form
All information will be kept confidential.
Insights
AI Use Cases Major Industries
Unlock the potential of AI for your business. Learn about its practical applications across industries in this comprehensive article.
Unlocking the power of AI for legal businesses: Use cases, benefits, and future trends
AI reshapes legal firms by automating tasks, enhancing research capabilities, and providing data-driven insights, promising efficiency and client-centric outcomes.
AI in predictive maintenance: A new era in industrial efficiency
AI offers numerous applications in predictive maintenance across a variety of industries, leveraging its capabilities in data analysis, machine learning, and predictive analytics to improve equipment reliability and operational efficiency.